We focus on developing practical Artificial Intelligence (AI) applications in challenging environments. ASK your daunting questions as we have the ability to harness research, data, and AI methodologies so you can explore the possibilities. We believe:
What can we do for you?
For starters, AI is generally defined as the theory and development of computer systems able to perform tasks normally requiring human intelligence type decision-making. Machine Learning is a type of AI in which computers use huge amounts of data to learn how to do tasks rather than being programmed to do them. Deep Learning is a type of machine learning in which neural networks discover non-linear, complex relationships from vast amounts of data.
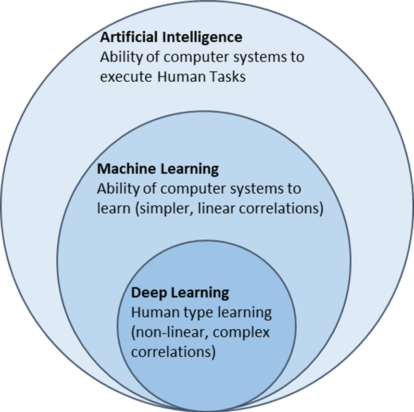
We caution that for suitability, beyond the buzzwords, effective implementation requires knowledge of complex processes for appropriate data/model/visual application and tuning to the pertinent task. As we illustrated below, balance the expectations of the use case depending on the skill/experience level and potential task complexity.
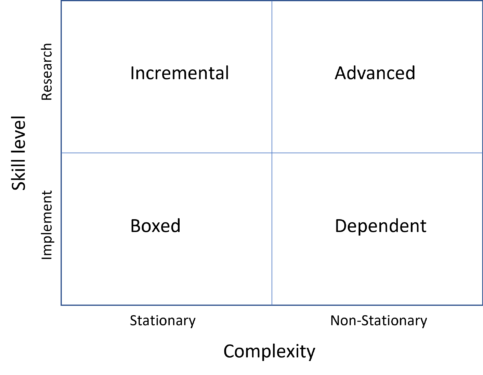
Within the financial markets, given the current AI business environment, one could consider using Natural Language Processing (NLP) techniques to read and file documents as somewhat of a boxed case. Whereas, implementing NLP techniques to read real time data for advantageous financial trading so far remains an advanced case. Another way to look at this is applying boxed cases in the non-stationary / research quadrant may be punitive and applying advanced cases in the stationary / implement environment may be overkill. Given our research and practitioner backgrounds we participate in all quadrants, however we are very focused on the Advanced quadrant as noted via our publications.
When done correctly, AI based decision support processes allow you to process numerous datasets, models, viewpoints, visuals, etc concurrently to continually assess historic and/or predicted data/performance within each aspect of the task, subject to defined or suggested objective functions.
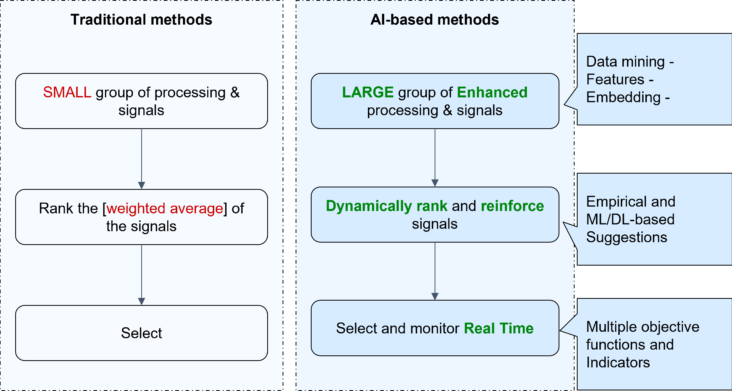
Implementing an AI based decision support system means that at a minimum you have additional data points to support your decision making. How could that be harmful?