With reference to the US AGG Mutual Funds, broadly speaking no. For the overall US AGG mutual fund market, assuming the Bloomberg US Agg Bond TR USD as the index, there is value in looking at historical price data (and the derived performance measures). As illustrated in Table 1, the overall market (without sampling) generated a 0.52 – 0.58% average annualized excess return over the Index (for each of 1, 2-, 3-, 5- or 10-year periods over the 12/31/2000 – 12/31/2022 evaluation period). With sampling, based on 40+ performance measures as illustrated in Table 2, there can be nearly 40% of the funds that are top quartile (depending on the performance measure used for selection and evaluation) that generated 0.9-1.7% average annual excess return (ranged 7.9% – (5.1)%) over the 1-2-3 year holding periods (here Excess Return as a selection criteria). However, high performance measure-based selection does not imply stable superior excess returns across all performance measures. Nor does it imply that it’s the same funds that are top quartile.
But,
1. Do you want the Index? The lower R2, high Alpha and low Beta of the Funds implies that the funds in general are not really in line with the Index (Bloomberg US Agg Bond TR USD). This also becomes evident from the peaks and troughs of the Excess Return and Relative Max Drawdowns, where the Index generated a better Sharpe over all of the assessment periods (see Table 1).
2 . Do the incremental ‘allocator/distributor’ fees erode the generated Excess Return? If the fees you pay are below 0.52% then as a market or via superior fund selection you may be better off (assuming Excess Return is your only objective function).
3. Which performance measures do you value more/less? Some performance measures have promising distributions as selection criteria and can support repeatability. The Explainability Index and Risk of Target (Image 1) gives a control panel for incorporating multiple performance measures.
4. Can you dig deeper for fund level granularity? This is insightful for assessing funds that may be ‘better’ over time, during certain times/regimes, only in certain markets, against certain benchmarks, at time of entry/exit (given the demonstrated over/under performance), etc. As in Event and Feature Engineering (yes, this Insights piece is basic simpler historical analysis, but we point this out as these questions may be coming up and will be covered in the journey’s next pieces (where more data is injected and ML/DL is applied to beyond canned what ifs).
When you are being pitched over 7,000 mutual funds[1] (in the US alone), how do you know the selection motivations are aligned? Beyond the regulatorily mandated disclosures, the distributors/allocators generally point to the historical performance of the funds and/or forecasted performance under scenarios. This relies on two facets: (a) the benchmark(s) being considered, and (b) the performance measure being evaluated. In this Insights piece, we look at a popular benchmark for the particular Asset Class and the fund performance against that benchmark as measured by 40+ performance measures estimated on a historical basis. Since evaluating so many performance measures can be unwieldy, we also assess the performance via the performance measures unifying framework of Explainability Index (EI) and Risk of Target (RoT)[2].
Data
We filter the US mutual fund data that are categorized as US Aggregate (US AGG), were at least 3 years old (considering 12/31/2000 – 12/31/2022 evaluation period), had over $ 1 billion in AUM and we evaluated the oldest share class. This filtering resulted in 168 funds in 2022 (with a range of 45-269 funds filtered for the analysis over the evaluation period).
Analysis
As a reminder, this Insights piece is the first part of the journey, where both the assessment and evaluation are based on historical price data (and derived performance measures) for both fund and benchmarks. Refer to the Explainability Index paper in footnote 2 for the methodology used for estimating the performance measures.
US AGG Market
Although it is difficult (or irrational) to invest in all the funds, it is important to look at the entire market as you never know the performance of the specific fund you have invested in will be (so at a minimum it sets the overall expectations). Therein the point here is to give a datapoint without selection bias for the entire market (as filtered for the Asset Class above), where the alternative is to invest in the Index (directly or via a proxy).
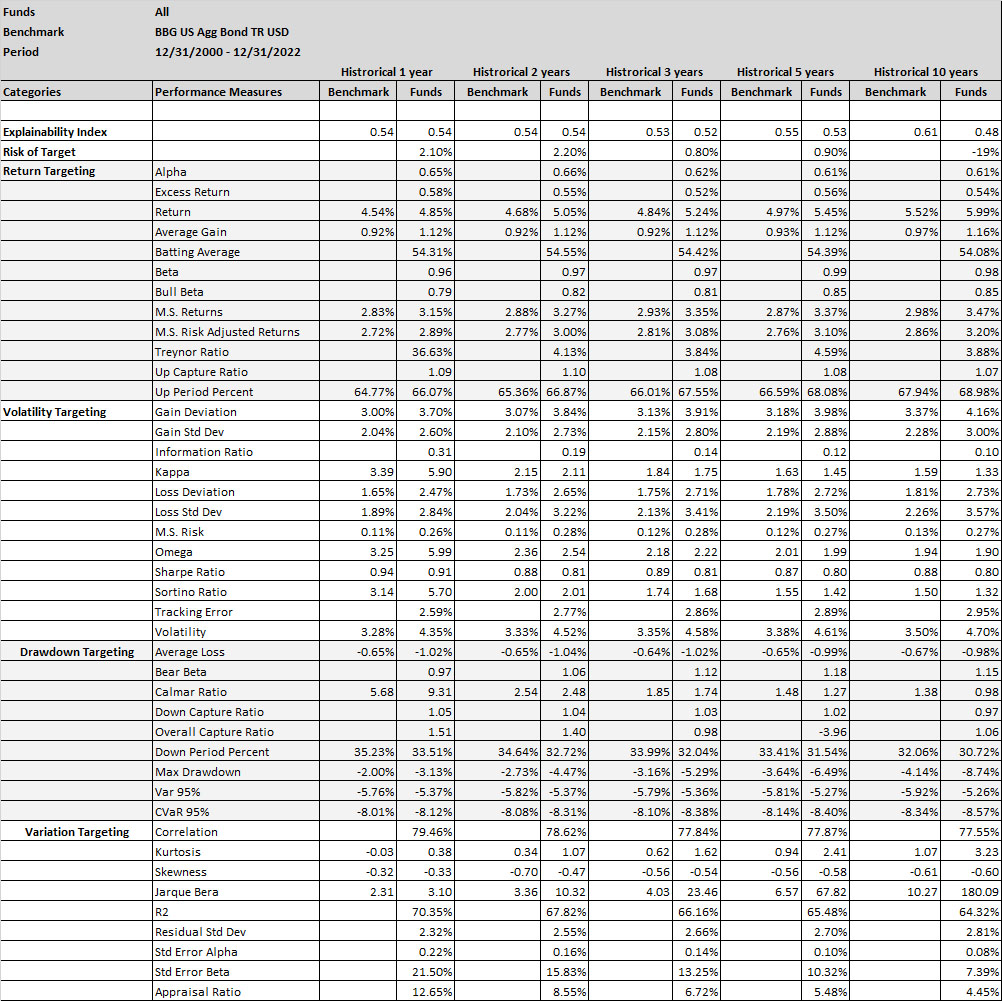
A simpler and explainable way to digest all the performance measures in Table 1 is to look at the Explainability Index Frameworks[3] presented below. This highlights the performance measure facets of the Funds that are better or worse than the designated Index.
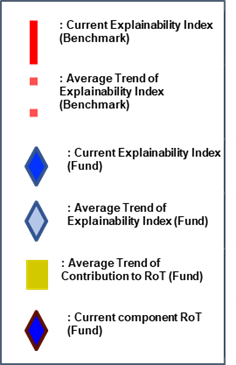
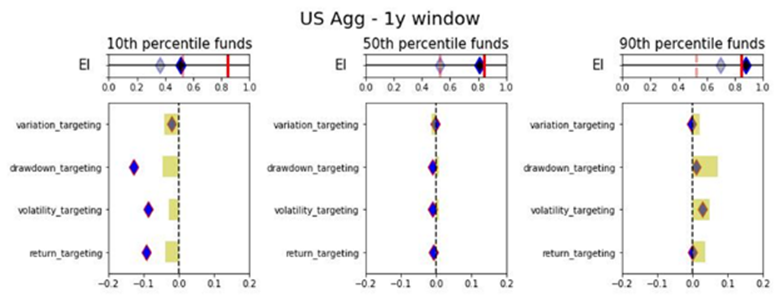
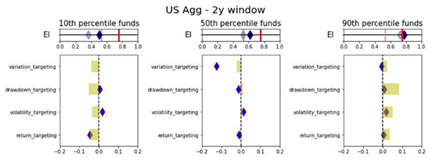
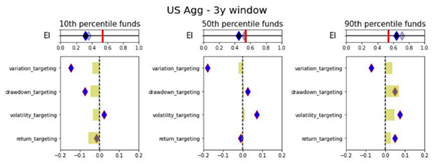
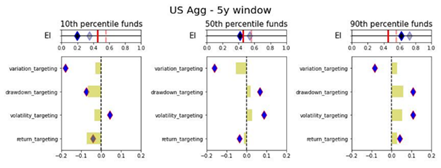
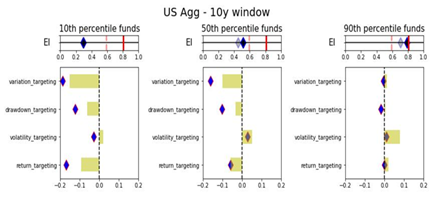
The Explainabilty Index bridges the final engineering jargon to illustrate and/or manage the performance measures as a control panel per what is important for the selector/allocator.
As illustrated in Figures 1, 2 and 3, the number of Funds that have a higher return than the benchmark is extremely volatile. Averages over the 20-year period for the Asset Class show that 56-62% of the funds beat the Index, which is incredibly high. This itself is a unique maker for the market (as we will see when we look at other Asset Classes), but again this is average and the range is very wide.
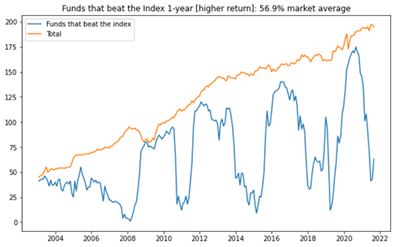
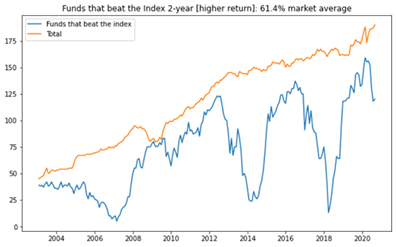
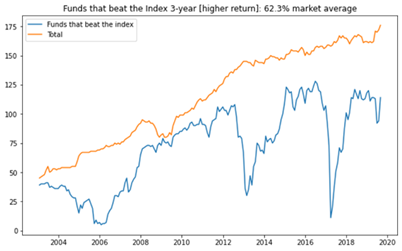
As an illustrative example, Figures 4 & 5 look more granularity at the two of the more widely assessed performance measures – Excess Return and Relative Max Drawdown (RMDD). In Figure 4. Excess Return exhibits periods of excessive over or under performance depending on the historic window. Overall, shorter term historic periods show a lot of cyclicality and longer-term periods are more consistent as in general the funds do better than the index from a return perspective.
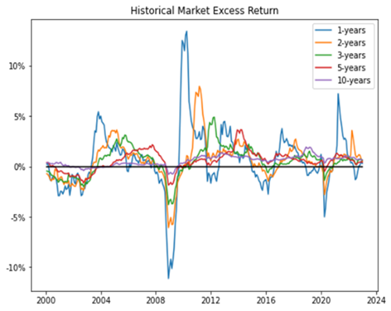
In Figure 5 the relative MDD shows extended periods of underperformance. This is not surprising as MDD is sticky given the nature of the look back periods. Funds in general have a higher MDD than the index in Table 1.
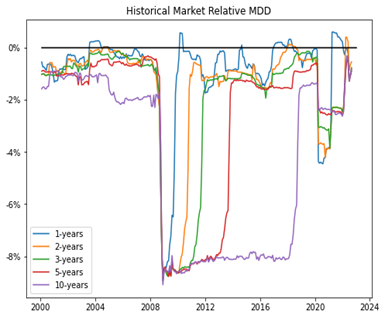
In Figure 6, we see that the funds have a relatively low R2 implying that the managers may not really be in line with the Index (especially over longer periods). That may also explain the higher Excess Return in Table 1 and the high outperformance averages in Figures 1, 2 and 3.
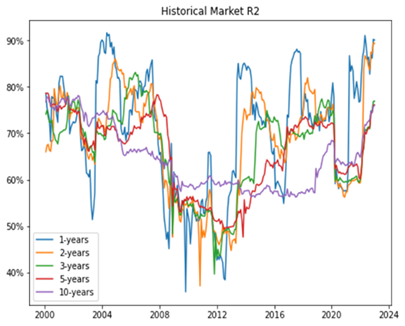
US AGG Market > Fund Selection (Rolling)
Within the broader market, we try to identify funds that may ‘in the future’ outperform the market given a particular objective function. For example, as illustrated in Table 1, if the market can generate Excess Return over the Index (albeit with lower Sharpe) the question becomes how good a predictor is that performance measure itself (or others) as a marker for identifying individual funds that have a higher probability of outperformance for that objective function. Further, since we cannot time the entry/exit we conduct the analysis on a rolling basis, where we use every month as a starting point for selection and ending point for the holding period. Final numbers are based on averages across the funds/months. It should be noted that the Tables in this Insights piece have a lot of embedded granularities (some of which we have tried to highlight in the Figures), but all are available upon request. With reference to the overall Insights journey, the selectors/allocators that are somewhat uncomfortable with the more advanced financial engineering methods and/or jargon (or have limited alternative data access) will reside on a spectrum here by using some or a combination of the performance measures covered in Table 1 as their selection and evaluation criteria.
Herein, many studies have been conducted on methods of selecting the ‘better’ performing funds via leveraging various lenses. In this Insights piece, we remain focused on only using historical fund and benchmark performance data for trying to identify the ‘better’ performing funds. Further, to keep this practical (as in easily implementable), we assess performance over set holding periods (for 1, 2- or 3-year periods), without rebalancing (or frequent trading) and measured across each of the performance measures as objective/evaluation criteria (versus some x factor (or such) model to assess alpha or other higher order value add).
As a framework, since the allocation can be made at any time, the analysis in Table 2 is based on rolling performance assessment. Where, every month, we take the top decile funds based on the historic performance measure (for each of 1, 2-, 3-, 5- or 10-year periods) and then evaluate the percentage of funds remaining as the top decile selection at the end of the Investment Period (or 1, 2- or 3-years forward). Procedurally for
- Selection, we take the top quartile funds for the historical performance of each measure (for each of 1, 2-, 3-, 5- or 10-year historical periods) and hold them for each of the investment periods (for 1, 2- or 3-year holding periods). This is done on a monthly basis over the entire evaluation period so, depending on the performance measure the fund selection can change.
- Objective/evaluation – for each month, at the end of each investment period (for 1, 2- or 3-year holding periods), we calculate how many of the initially selected funds remain a top fund based on the same performance measure. We also evaluate if the selected funds remain a top fund based on all of the other performance measures. Table 2 illustrates results for Alpha (Higher), Excess Return (Higher) and Return (Higher) as the objective/evaluation criteria (where the results of all other categories and performance measures are available upon request).
Final percentages for the objective/evaluation are based on the averages. Note, over the evaluation period (from 2000-01-31 to 2022-12-31) if the fund had a track record lower than the historical evaluation period, the measure was evaluated from its inception.
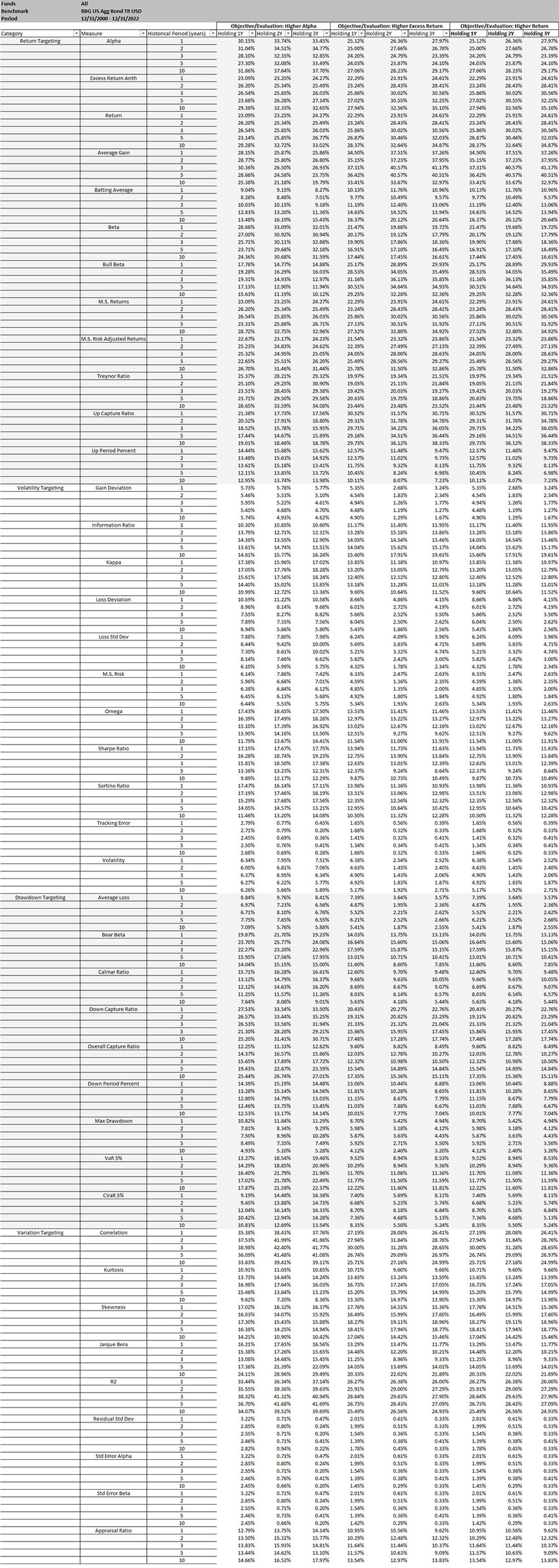
Overall depending on the performance measure selected there can be an up to 42% chance of being in the top decile (under certain selection criteria, evaluation criteria and investment period). This is a remarkably high and unexpected number, but we again point to the framework setup and its specific application to the US AGG market. Further, it should be noted that it does not imply that it is the same funds that remain top quartile. It should be noted that using a combination of fixed performance measures and weights should generally give results within the performance measure ranges.
For a more granular analysis, let’s assess the historic Excess Return as a predictor. As a reminder, the results of all other performance measures are available upon request. From Table 2, assuming we would have invested in the top decile funds as classified by the historic 3-year analysis, where Figure 7, 8 and 9 give the performance of those funds after 1, 2- and 3-year holding periods. Note, average stands for investing equal weights in all of the identified top decile funds, worst stands for picking the worst fund in the top decile every time, and best stands for picking the best fund in the top decile every time.
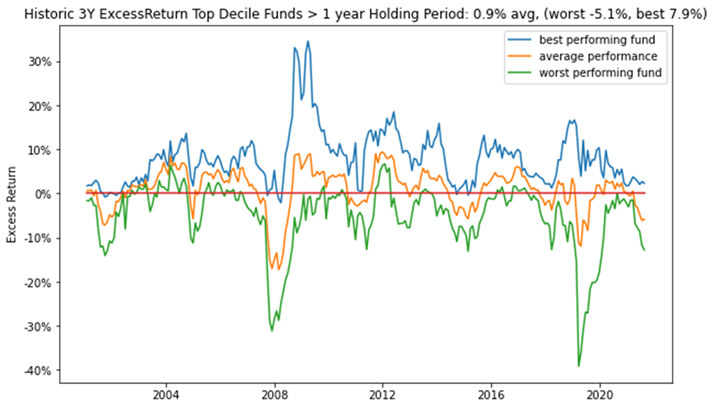
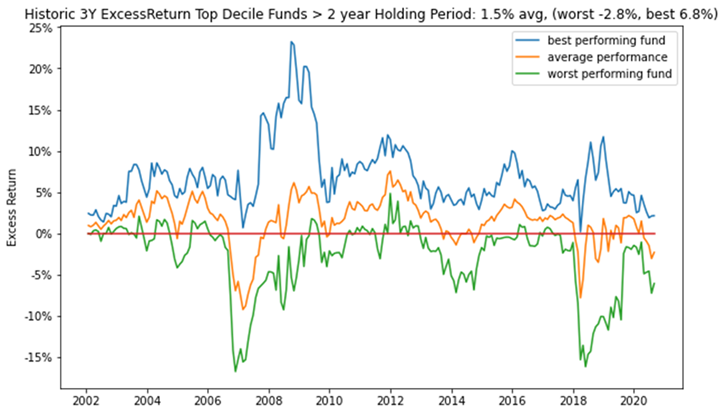
On average, we find that using just historic (3-year) Excess Return as a selection and evaluation criterion for the increases the selection Excess Return value from 0.52% to 1.7% and similarly for other periods (assuming impact on other performance measures are not considered).
If there is implied value in generally using the performance measures, then the question becomes if any of the performance measures show unique markers that make them better qualified for the selection. As illustrated in Figures 10, 11 and 12, we compare the distributions of funds that generated Excess Return over the index (at the end of the investment periods) with the funds that did not beat the index. Figures 10, 11 and 12 show that Excess Return distributions show it not to be a clear discerning marker.
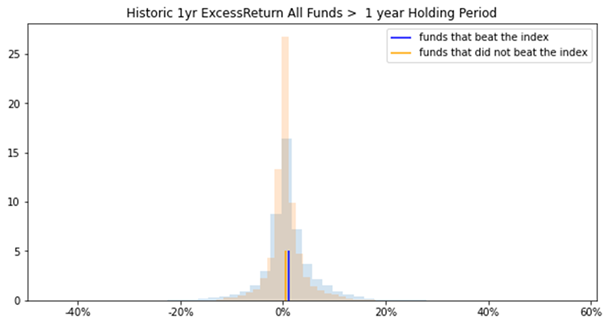
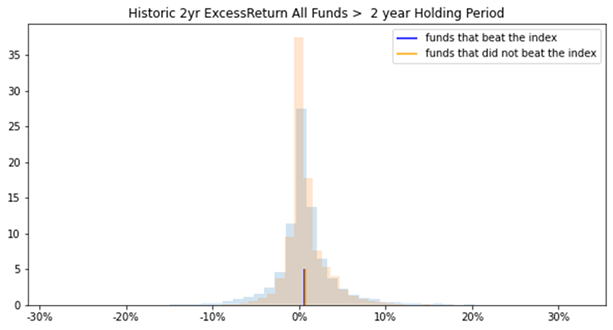
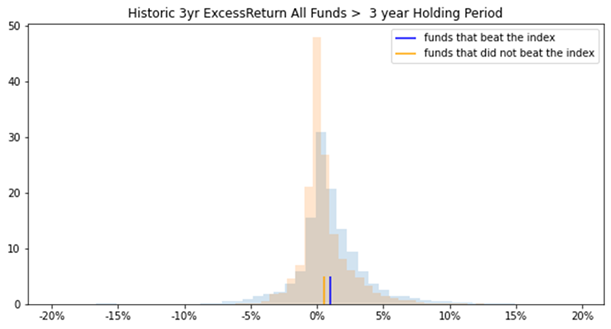
In assessing the distribution profiles of all performance measures listed in Table 1 we isolate the ones that exhibit more pronounced differences (as illustrated in Figures 13, 14, 15 and 16). For example, as illustrated in Figure 13 and 14, funds with a lower R2 seemingly were more likely to beat the Index. Where as in Figures 15 and 16 show Average Gain as a unique marker also.
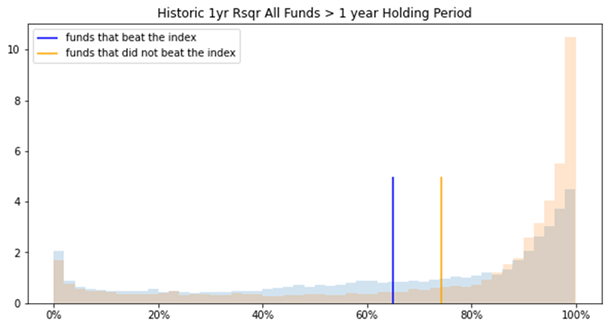
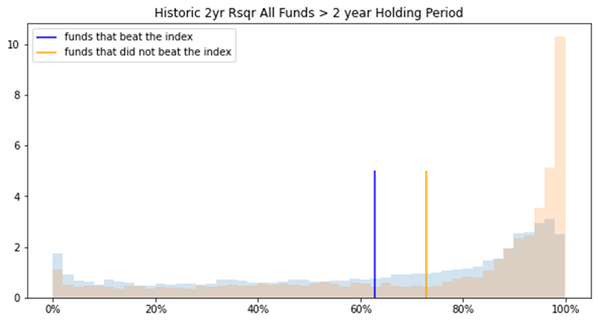
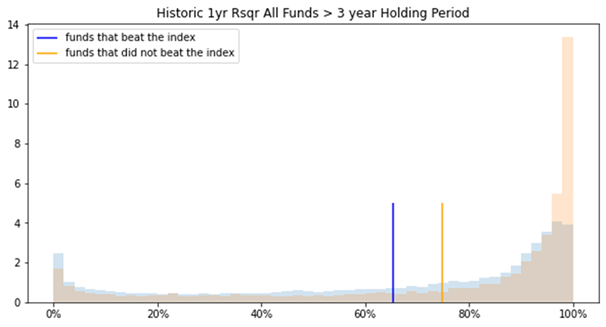
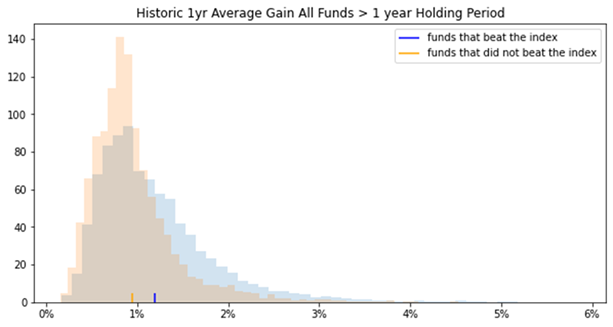
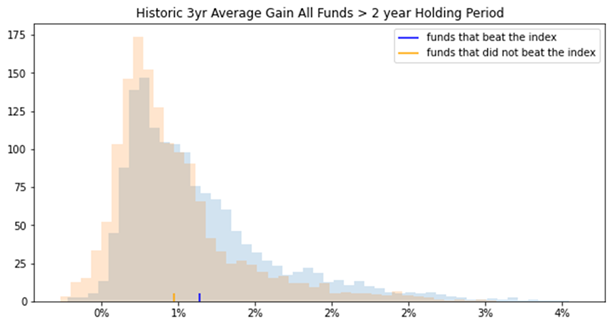
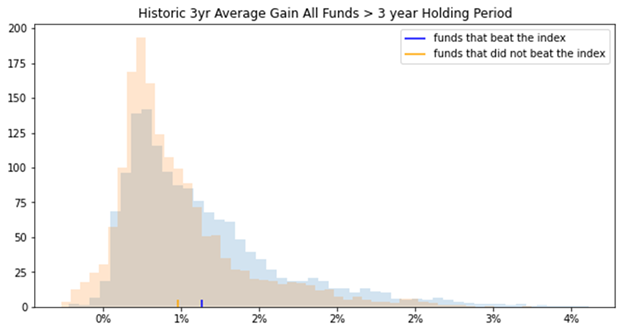
As an illustration, since we find R2 to have unique marker, we assess the Excess Returns based on using the 3-year historic R2 performance as a selection criteria (Figures 19, 20 and 21). It reflects a tighter spread than using Excess Return and average seems to improve over the short time period.
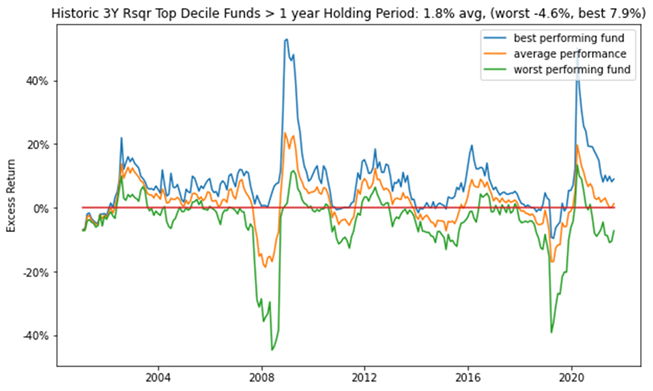
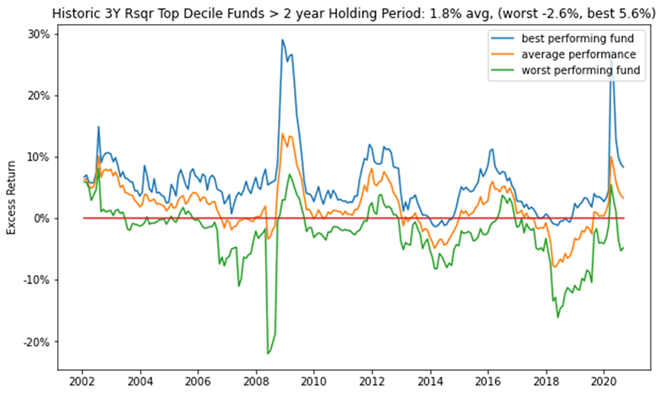
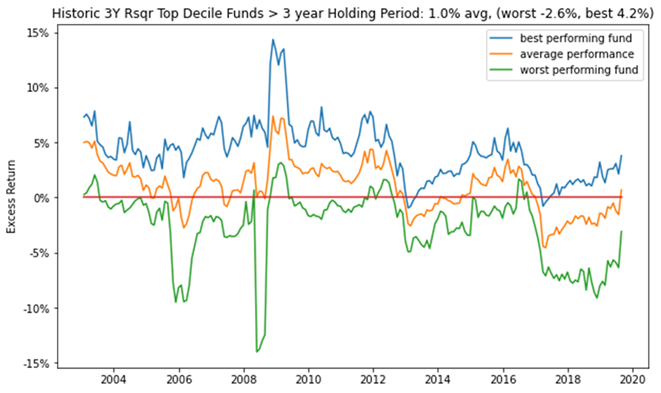
US AGG Market > Fund Selection (point in time)
Looking at monthly rolling performance statistics has lots of embedded nuances, statistics and in general can be overwhelming. In is section, we do a point in time analysis, where we assume that the decisions were made on 2018-12-31 to select the top decile funds based on the 3-year historic Excess Return and we assess the Total Return of the funds in 2019, 2020 and 2021 (as illustrated in Figures 22, 23, 24 and 25).
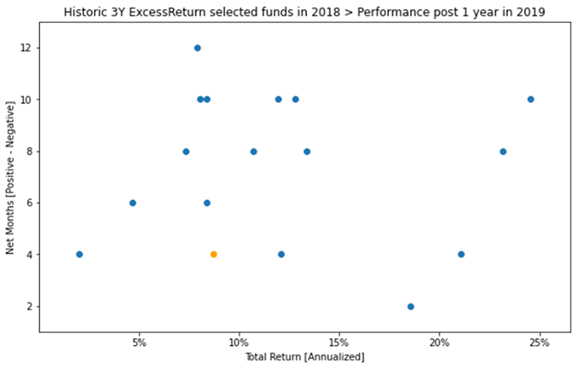
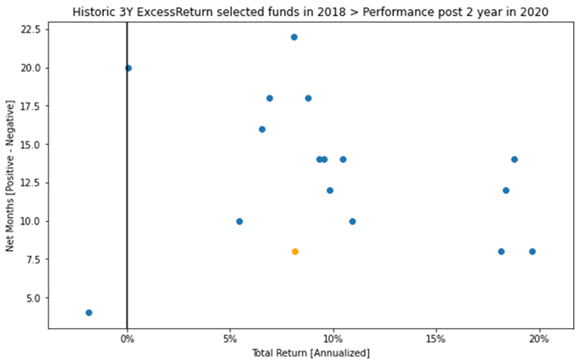
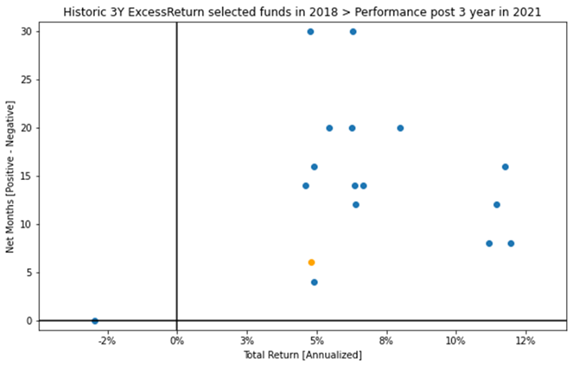
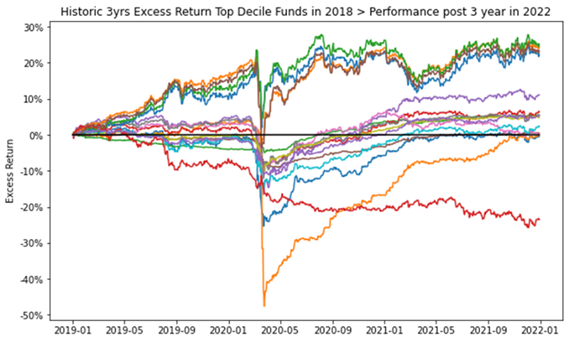
Future performance of the top decile for funds selected on 2018-12-31 according to Excess Return Ranking (Figures 26, 27 and 28). As with Figure 25, over the 3-year period the funds normalize beyond the short-term volatility to trend back to the Excess Return quadrant. Figures 26, 27 and 28 assess the Excess return and net positive/negative returns of the previously selected top quartile funds in the future 1, 2 and 3 year periods.
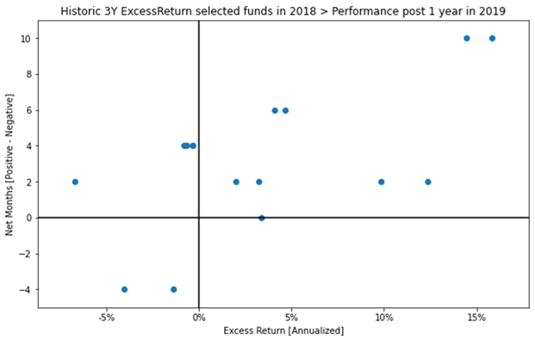
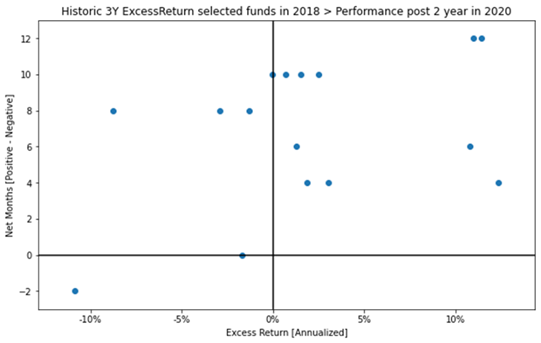
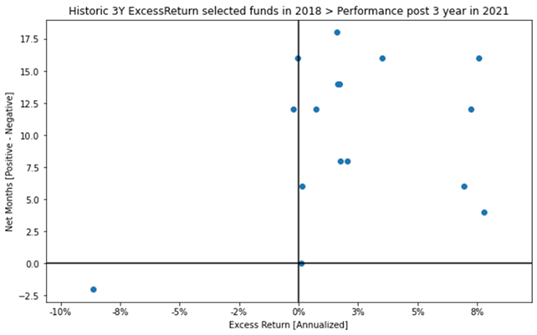
Figure 29 looks at 1 year cumulative return profile funds in the positive quartile of the funds in Figure 26. And, Figure 30 for the funds in Figure 27. Over shorter periods the performance expectations are more volatile.
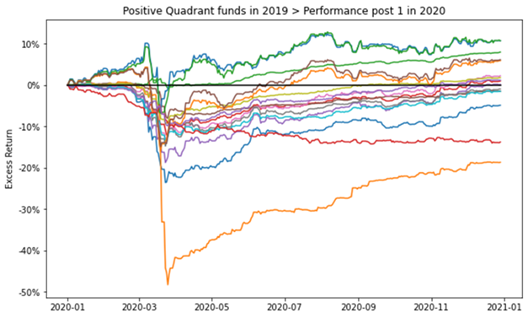
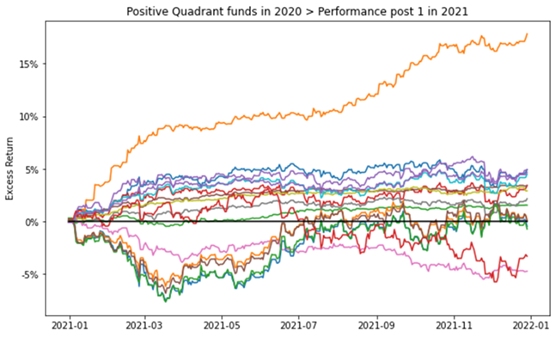
As highlighted above the historic analysis for the US AGG market seems to show promise when Bloomberg US Agg Bond TR USD is the index. However, deriving true value requires a lot of what ifs for isolating feature and event importance as points of entry/exit can dramatically impact the results due to the volatility shown in Figures 1, 2 and 3. The What ifs can be an anecdotal or iterative process as is expected from a fundamental type analysis.
Contact us for information about a particular fund, performance measure, time period, etc.
Email: info@ask2.ai for questions.
Next
Insights 2.11. Mutual Fund Manager Selection – Basic Historic Analysis: Are you always wrong?
Focus: US Large Cap Mutual Funds (US LC)
Background
Insights 2.00. Mutual Fund Manager Selection – Setting up the framework
Insights 2.10. Mutual Fund Manager Selection – Basic Historic Analysis: Are you always wrong?
We begin by holistically looking at the US mutual fund manager landscape from a historical fund price perspective and assess the ability of widely used performance measures for manager selection. This is done both at the market and individual fund level. We look at simple back testing and predefined assessments. We will give Insights for every Asset Class. This was focused on US AGG. Next: US Large Cap.
Insights 2.20. Mutual Funds – Is there value in leveraging larger datasets?
We incorporate larger volumes of macro data, market data, performance measures, holding data, alternative data, etc. We introduce forms of feature engineering to generate signals for regimes, factors, indicators and measures using both raw and reduced datasets. We also introduce synthetic data generation to supplement sparse datasets.
Insights 2.30. Mutual Funds – Machine and Deep learning edge?
We incorporate evolving market conditions, performance measures, weights, events, predictions, etc. by leveraging Machine Learning techniques for real time and simulated multivariate analysis. Then we allow the system to do feature and event engineering by assessing various Deep Learning methods.
Extensions can be drawn to other types of managers, assets and markets. Here we will stay at the framework level, but will refer to our other papers that delve into the technical nuances and discoveries. Additionally, we will share similar series of Machine and Deep Learning framework papers for other aspects of the Investment lifecycle – asset allocation, portfolio management, risk management, asset planning, product development, etc.
These are all underpinnings of our Platform, where it is built to support any/all permutation/combination of data/models/visuals.
[1] 27,000+ if you assume all share classes. Also, not including SMAs, ETFs, etc.
[2] Hirsa, Ali and Ding, Rui and Malhotra, Satyan, Explainability Index (EI): Unifying Framework of Performance Measures and Risk of Target (RoT): Variability from Target EI (January 23, 2023). Available at SSRN: https://ssrn.com/abstract=4335455
[3] The current is based on annualized over a (x)yr period and the average is based on rolling the (x)yr window every year for 20yrs; we assess the fund/portfolio as of the last evaluation date rolled back. Analysis assumptions: Measures: Absolute. Time Variation: No. Threshold/Scale: Market Index. Categories: Yes. Weights: Equal. Type: Arithmetic. 50th percentile defined as 45-55%.