$816 billion invested and you are lucky if you get what you think you are buying! 43% of the funds have reclassified themselves (some more than 4 times), there is marginal prediction power in performance measures (less than 1 in 5 chances), performance has struggled to beat the Russell Mid Cap TR USD and yet, over the last decade the US Mid Cap mutual fund market grew 2.5x!
Overall, for the US Mid Cap mutual fund market, assuming the Russell Mid Cap TR USD as the index, there may be lesser value in looking at historical price data (and the derived performance measures) as selection criteria. As illustrated in Table 1, the overall market (without sampling) generated a 0.0 – 0.52% average annualized excess return over the Index (for each of 1, 2-, 3-, 5- or 10-year periods over the 12/31/2000 – 12/31/2022 evaluation period). With sampling, based on 40+ performance measures as illustrated in Table 2 there is less than 1 in 5 chance that the selection is in the top decile (depending on the performance measure used for selection and evaluation), but when used as a selection criteria they generated (0.8)% – (1.7)% average annual excess return (ranged 11.6% – (12.7)%) over the 1-2-3 year holding periods (here Excess Return as a selection criteria). Cannot be more random than this. Further note that high average performance measure-based selection does not imply stable superior excess returns across all performance measures or periods. Nor does it imply that it’s the same funds that are top quartile.
So,
1. You may want the Index. There exists little to no consistent relationship between the funds in the top percentiles of performance measures and the top percentiles of excess return. Moreover the degree of fund reclassifications make the Asset Class transitory in nature with lower reliability if the holding period is long.
2. Lack of Asset Class classification consistency. Throughout the evaluation period, many funds failed to qualify for the set Mid Cap [set criteria of having an AUM of over 1 bn, existed for 3 years and declared as a US Fund Mid-Cap Blend, US Fund Mid-Cap Growth, or US Fund Mid-Cap Value Categories]. Many funds changed their investment prospectus to Large Cap and other alternative categories. This leads to the question of what are you truly investing in when selecting Mid Cap funds, as in future periods these funds may fall out of your self determined qualifications for Mid Cap.
3. Lack of repeat performance. When analyzing the Mid Cap market on an individual fund level, at first blush, there exist certain funds which manage to beat the benchmark with respect to returns over some evaluation periods. However, investing in these is period dependent, where they outperformed during Covid but pre-Covid were sub benchmark. Additionally, when examining funds which consistently outperform the index, a majority of funds only manage to qualify as a Mid Cap mutual fund for a short period of time. Consequently, these funds appear to significantly drop in terms of performance when analyzing excess returns over the entire lifetime of the fund.
4. Low selection power of features/measures. Assessing the historical data there seem to be no discerning markers that can help pick the future better performers – at best 1 in 5. There exists little to no overlap between funds that consistently beat the benchmark and while remaining in the top decile for each isolated performance measure. For some performance measures, some funds manage to routinely remain in the top decile while other measures see the top performers spread out over a wider set of funds. While consistency is a good trait in the fund selection process, the measures with the most concentrated group of top performers may see a drastic dissimilarity between historical and future performance as even those top funds have a wide performance spread in Excess Return. The Explainability Index and Risk of Target (Image 1) gives a control panel for incorporating multiple performance measures.
5. No value of additional fees. Do the incremental ‘allocator/distributor’ fees erode the generated Excess Return? If the fees you pay are below 0.50% and you hold the asset for 5+ years, then as a market or via superior fund selection you may be marginally better off (assuming Excess Return is your only objective function), but as we see in the analysis that is a tall order.
6. Can you hunt Unicorns? This quest may be the factor keeping the investors in the Asset Class. We have a crystal ball to find funds that may be ‘better’ over time, during certain times/regimes, only in certain markets, against certain benchmarks, at time of entry/exit (given the demonstrated over/under performance), etc. As in Event and Feature Engineering (yes, this Insights piece is basic simpler historical analysis, but we point this out as these questions may be coming up and will be covered in the journey’s next pieces (where more data is ingested and ML/DL is applied to beyond canned what ifs)). At first glance it may be fleetingly possible, but only if you caught them early or during the beginning of a regime. But, if timing is not possible it may not be worth being exposed to the fund level risks.
When you are being pitched over 7,000 mutual funds1[1] (in the US alone), how do you know the selection motivations are aligned? Beyond the regulatorily mandated disclosures, the distributors/allocators generally point to the historical performance of the funds and/or forecasted performance under scenarios. This relies on two facets: (a) the benchmark(s) being considered, and (b) the performance measure being evaluated. In this Insights piece, we look at a popular benchmark for the particular Asset Class and the fund performance against that benchmark as measured by 29 performance measures estimated on a historical basis. Since evaluating so many performance measures can be unwieldy, we also assess the performance via the performance measures unifying framework of Explainability Index (EI) and Risk of Target (RoT)[2].
Note that since we want each of the Insights Historical Analysis pieces to be comparative and standalone, the framework and language is similar across the 2.10 Insights. We add some additional analytical color as we add new markets to highlight incremental optionality. As expected, the numbers and observations are market dependent.
Data
We filter the US mutual fund data that are categorized as US Mid Cap, were at least 3 years old (considering 12/31/2000 – 12/31/2022 evaluation period), had over $ 1 billion in AUM and we evaluated the oldest share class. This filtering resulted in 138 funds in 2022 with a range of 47 – 138 for funds filtered for the analysis over the evaluation period).
Analysis
As a reminder, this Insights piece is the first part of the journey, where both the assessment and evaluation are based on historical price data (and derived performance measures) for both fund and benchmarks. Refer to the Explainability Index paper in footnote 2 for the methodology used for estimating the performance measures and all other calculations.
ALL US MID CAP FUNDS / MARKET
Although it is difficult (or irrational) to invest in all the funds, it is important to look at the entire market as you never know the performance of the specific fund you have invested in will be (so at a minimum it sets the overall expectations). Therein the point here is to give a datapoint without selection bias for the entire market (as filtered for the Asset Class above), where the alternative is to invest in the Index (directly or via a proxy).
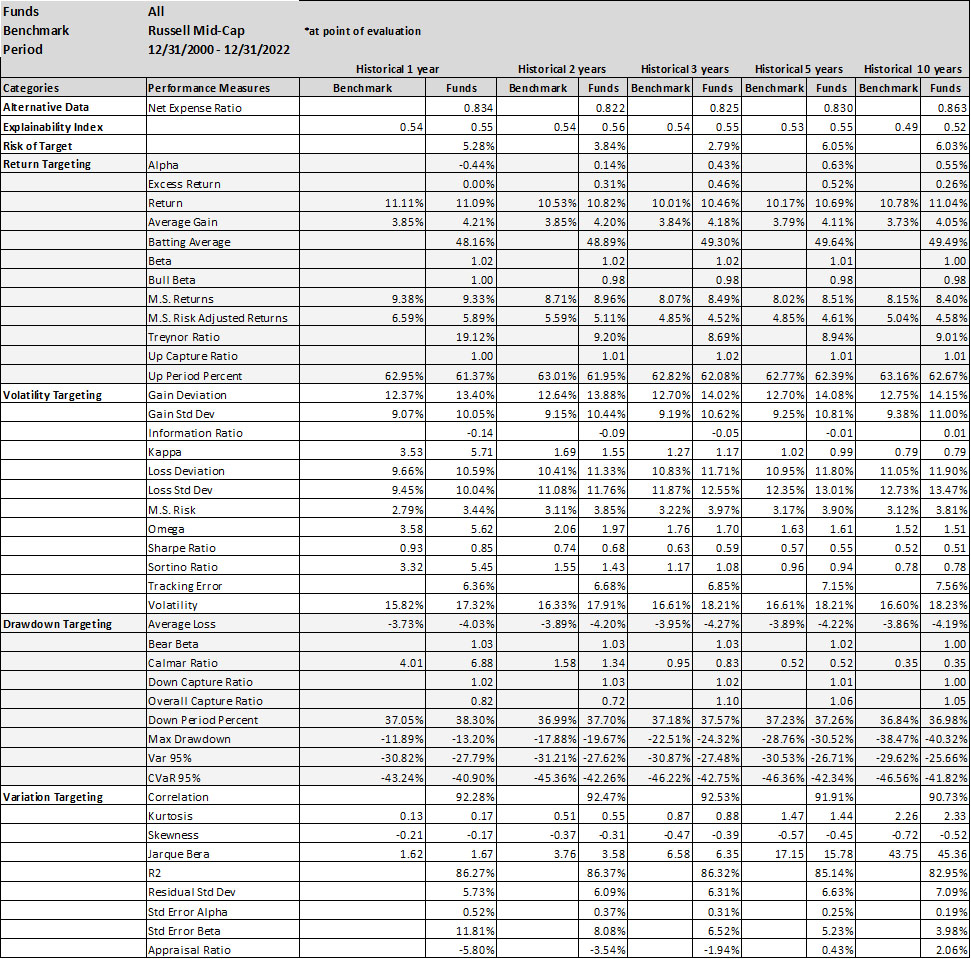
A simpler and explainable way to digest and explain all the performance measures in Table 1 is to look at the Explainability Index Frameworks[1] presented in Illustration 1. The framework highlights the performance measure facets of the Funds that are better or worse than the designated Index. Table 1 will largely correspond to the 50th percentile funds, where the performance is overlapping. The performance of the top quartile will correspond to Table 2, where the selection criteria can yield better performance. However, unlike Table 2 where the measure has 100% of the weight, here all categories are given a 25% or equal weight. Think of the Explainability Framework as a doctor’s report with the thermometer/temperature being the first order of assessment, followed by a set of category indicators of vitals. So, are you worse off than the target (as in more temperature than normal) and why (as in which measures are indicating deviations)?
Illustration 1. Explainability Framework
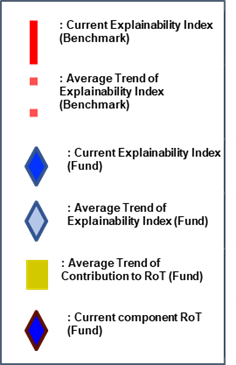
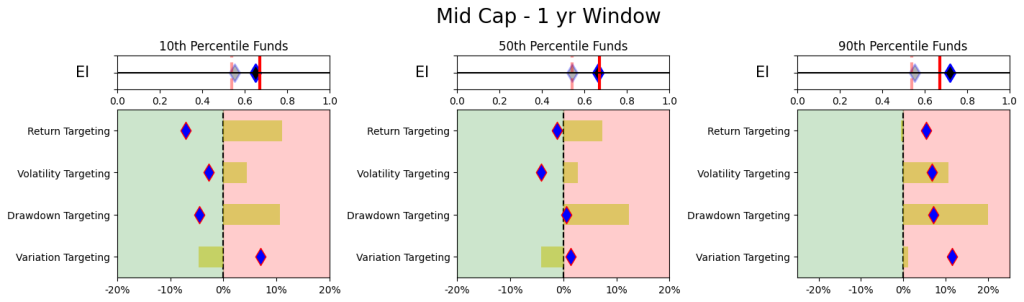
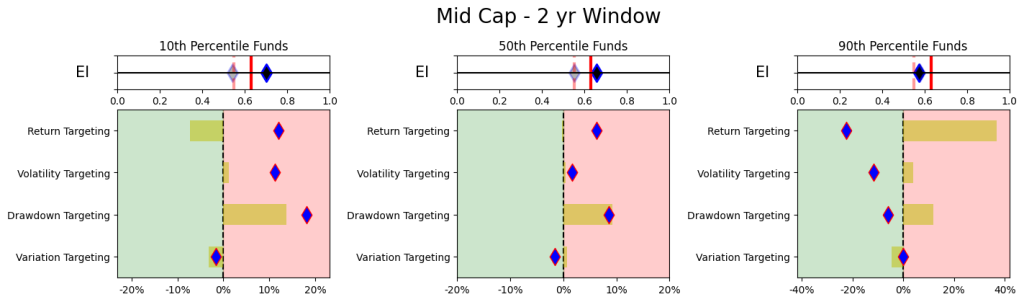
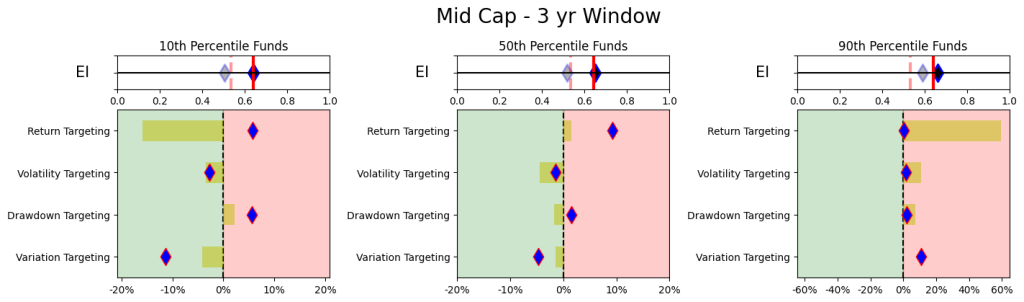
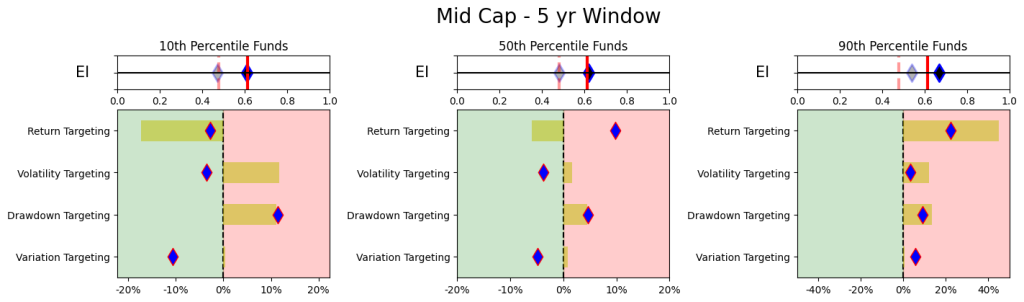
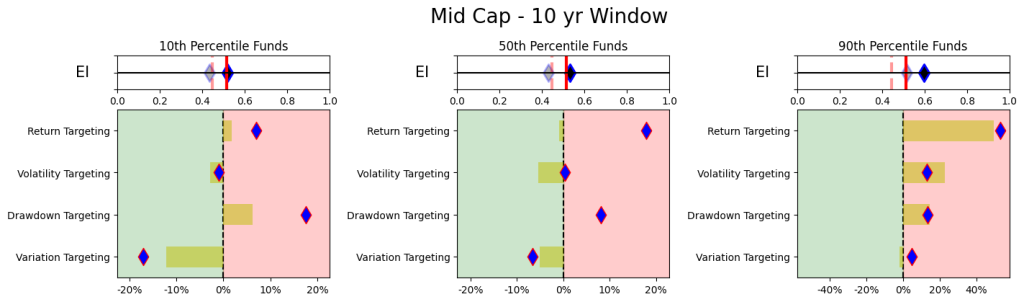
The Explainability Framework bridges the final engineering jargon to illustrate and/or manage the performance measures as a control panel per what is important for the selector/allocator. More on its usages in Insights 2.01 – https://www.ask2.ai/insights-201/
As a market, as illustrated in Figures 1, 2 and 3, the number of Funds that have a higher return than the benchmark is extremely volatile. Averages over the 20-year period for the Asset Class show that 36 -40% of the funds beat the Index. However, when you break the periods into parts then, until 2011 you had ~34% of the funds doing better, but since then the number has increased to a ~36% average (for the 1-,2- or 3-year hold periods). Whereas, the current NAV of funds (across all share classes) that beat the index was $308 bn (with total NAV of $816 bn) in 2022 versus NAV of $194 bn of the funds that beat the index in 2011 (with total NAV $ 371 bn).
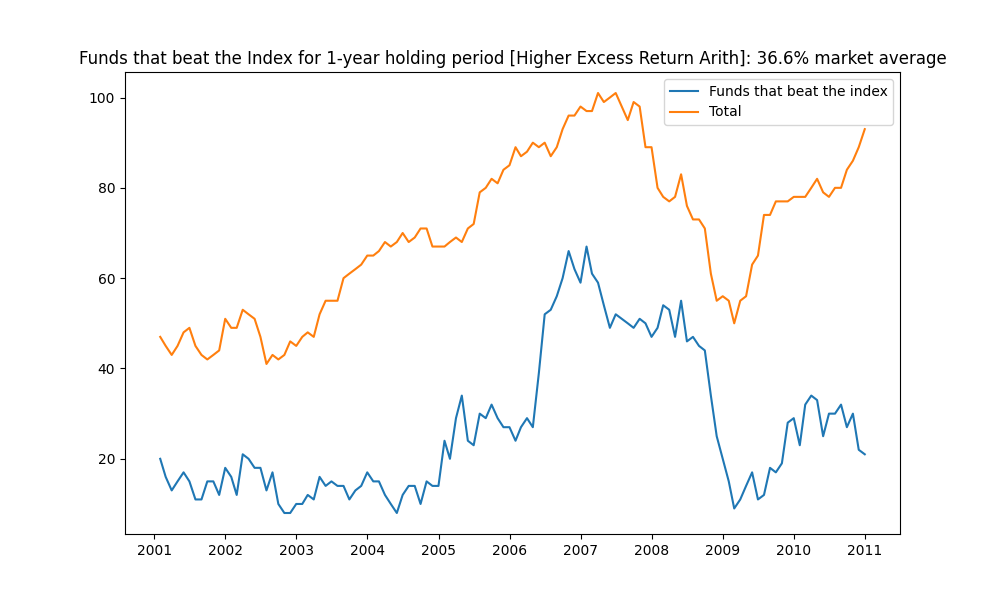
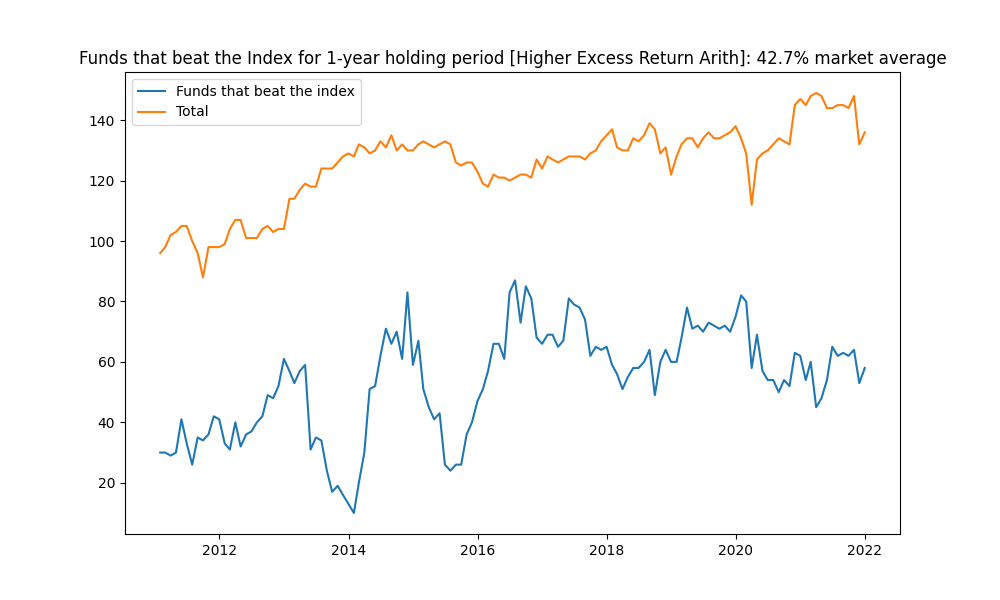
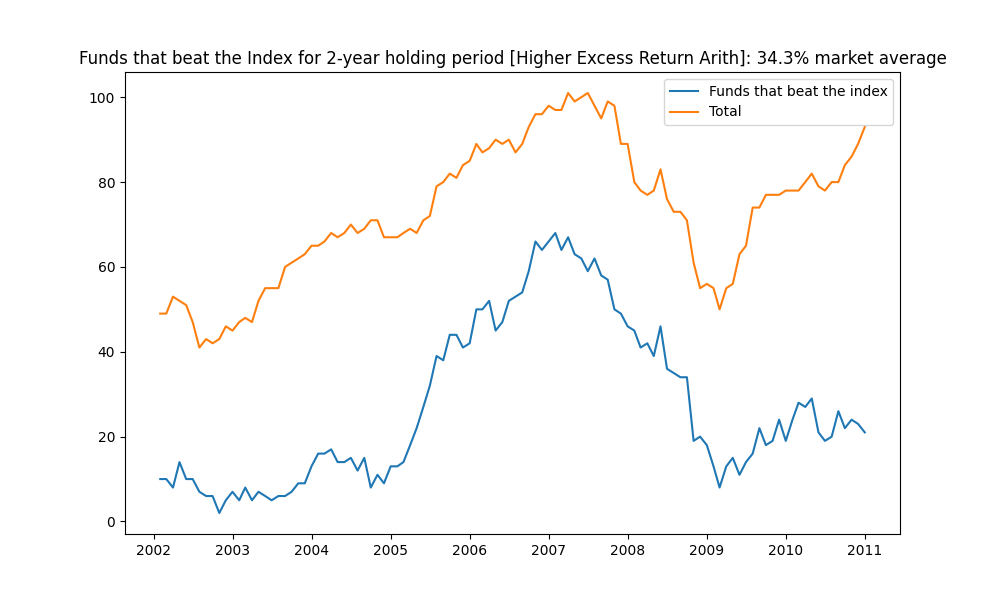
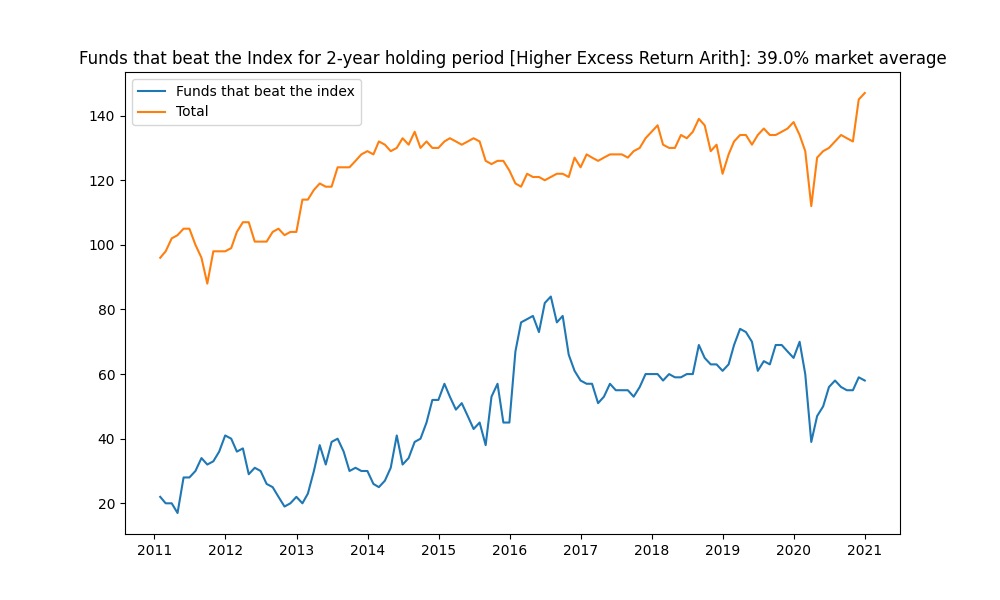
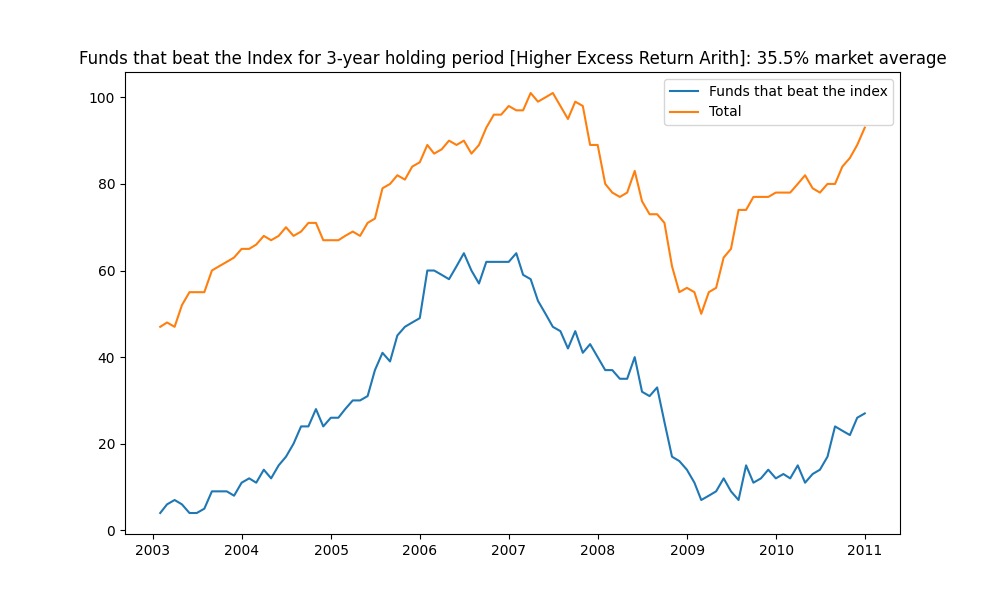
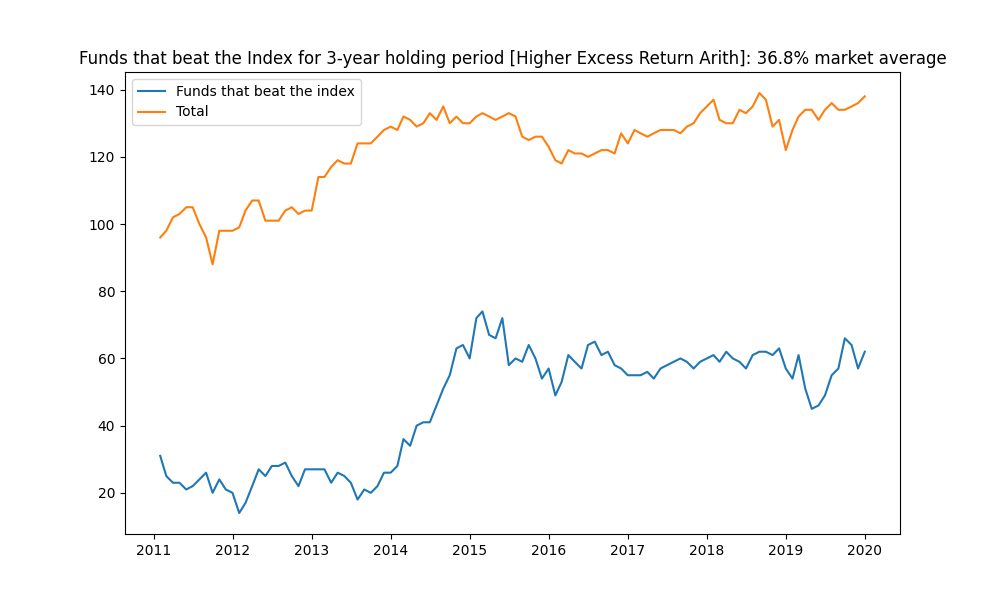
One major issue with the Mid Cap Asset Class is the reclassification by funds. As noted in Figures 4 and 5, a large portion of the funds are re-classifying themselves. Figure 4 illustrates the type of reclassifications and Figure 5 illustrates the number of times the fund reclassified itself. If you are not a short-term investor then this raises questions about reliability and sustenance.
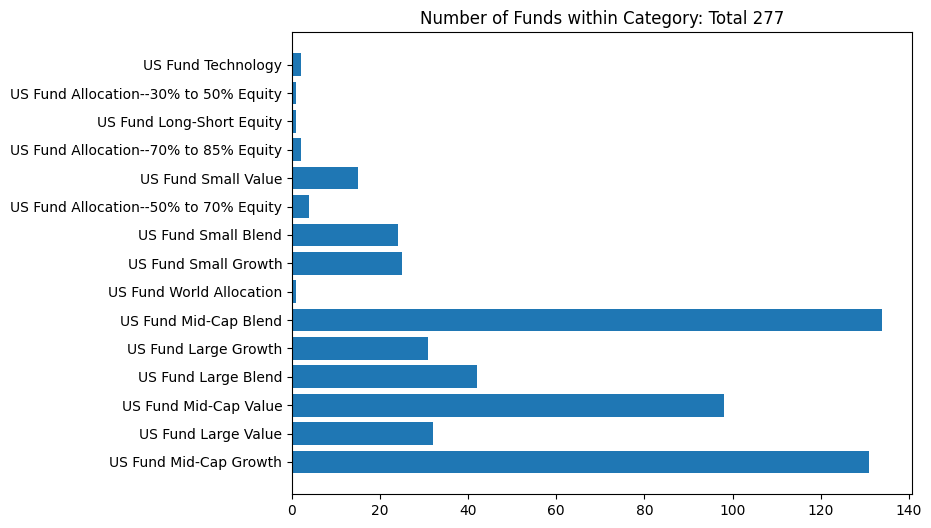
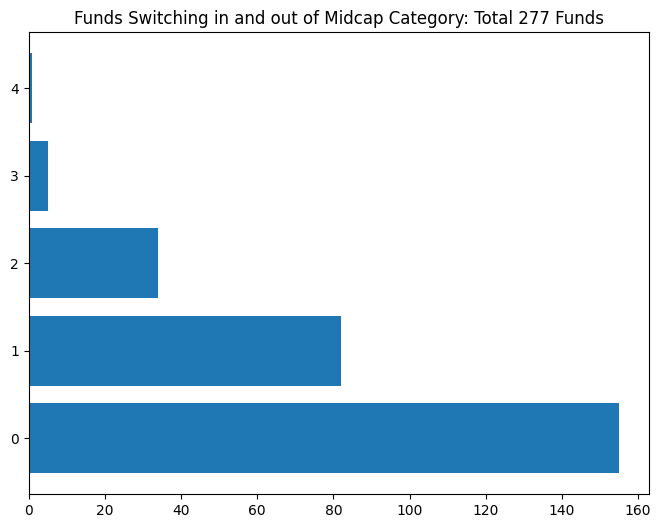
From Table 1, Figures 6 & 7 look more granularly at the two of the more widely assessed performance measures – Excess Return and Relative Max Drawdown (RMDD). Figure 6. Excess Return exhibits periods of excessive over or under performance depending on the historic window. Overall, shorter term historic periods show a lot of cyclicality and longer-term periods are more consistent. However, in general, the US Mid Cap funds over the last decade have largely done a poorer job than the Index.
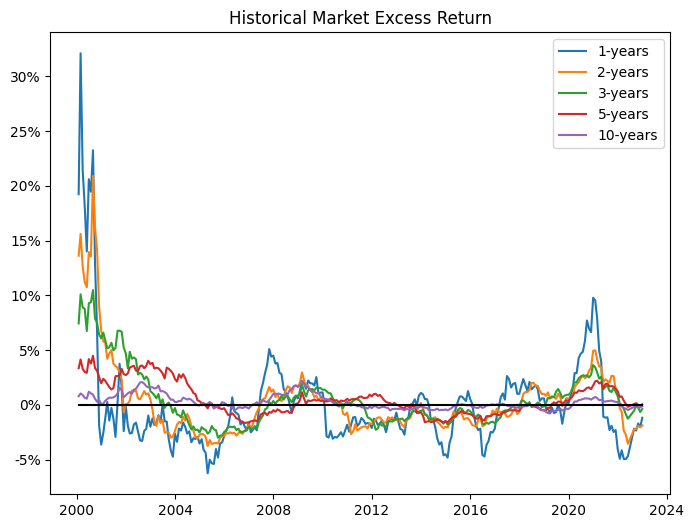
In Figure 7 the relative MDD shows more volatility especially in the pre-2011 and Covid periods, where the funds outperformed the Index and then dropped significantly. More recently, as also seen in Table 1. the relative MDD has been poorer than the Index.
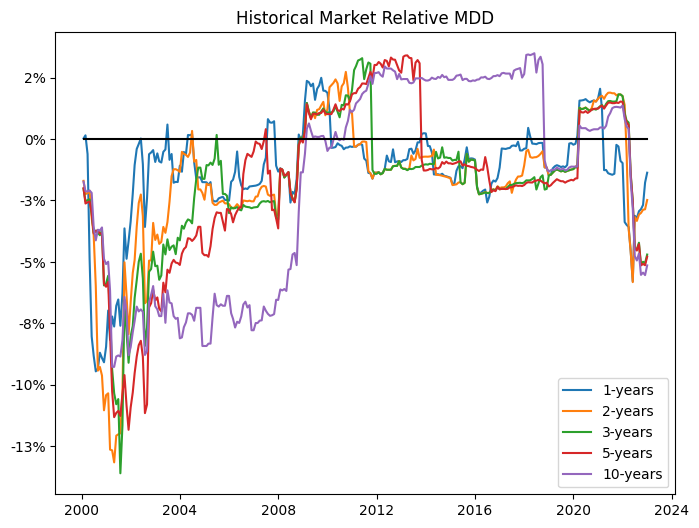
In Figure 8, we see that the funds have a relatively higher R2 implying that the managers are in line with the Index over shorter periods. As in Table 1. this is the lower Excess Return and high Beta in the overall market.
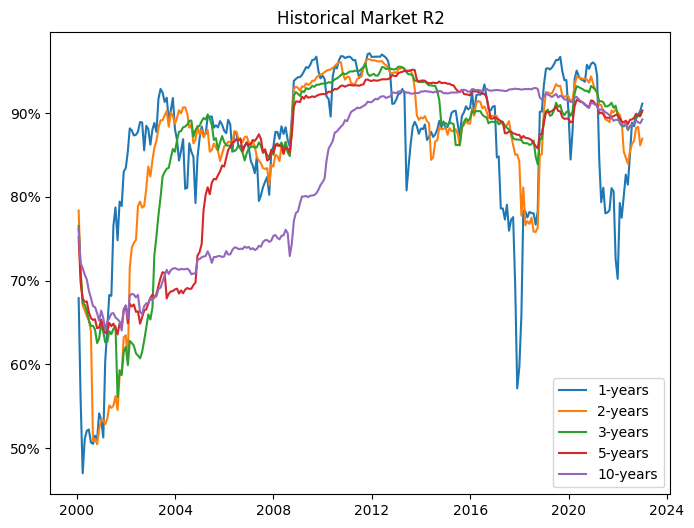
US MID CAP FUNDS > SELECTING BETTER PERFORMERS (ROLLING BASIS)
Within the broader market, the task becomes to try to identify funds that may ‘in the future’ outperform the market given a particular objective function. For example, as illustrated in Table 1, if the market can generate marginal Excess Return over the Index (albeit with lower Sharpe) the question becomes how good a predictor is that performance measure itself (or others) as a marker for identifying individual funds that have a higher probability of outperformance for that objective function.
Herein, many studies have been conducted on methods of selecting the ‘better’ performing funds via leveraging various approaches. In this Insights piece, we remain focused on only using historical fund and benchmark performance data for trying to identify the ‘better’ performing funds. To assess the feature importance we look at the performance measures as predictors from three perspectives –
- Measure based
- Distributional Shifts
- Regression
To keep this practical (as in easily implementable), we assess the performance over set holding periods (for 1, 2- or 3-year periods), without rebalancing (or frequent trading) and as measured across each of the performance measures as objective/evaluation criteria (versus some x factor (or such) model to assess alpha or other higher order value add). Further, since we cannot time the entry/exit we conduct the analysis on a rolling basis, where we use every month as a starting point for selection and ending point for the holding period. Final numbers are based on averages across the funds/months. It should be noted that the Tables in this Insights piece have a lot of embedded granularities (some of which we have tried to highlight in the Figures), but all are available upon request. With reference to the overall Insights journey, the selectors/allocators that are still exploring the more advanced financial engineering methods and/or jargon (or have limited alternative data access) will reside on a spectrum here by using some or a combination of the performance measures covered in Table 1 as their selection and evaluation criteria.
A. Measure based
The first approach becomes using each of the performance measures as a selection criteria for the funds. As a framework, since the allocation can be made at any time, the analysis in Table 2 is based on rolling performance assessment. Where, every month, we take the top decile funds based on the historic performance measure (for each of 1, 2-, 3-, 5- or 10-year periods) and then evaluate the percentage of funds remaining as the top decile selection at the end of the Investment Period (or 1, 2- or 3-years forward). Procedurally for
- Selection, we take the top quartile funds for the historical performance of each measure (for each of 1, 2-, 3-, 5- or 10-year historical periods) and hold them for each of the investment periods (for 1, 2- or 3-year holding periods). This is done on a monthly basis over the entire evaluation period so, depending on the performance measure the fund selection can change. Note that results in Table 2 are one directional as we feel all the permutations/combinations could make the point illustration unwieldy (e.g., positive excess return is good, but it is similarly possible that negative excess return is a better marker).
- Objective/evaluation – for each month, at the end of each investment period (for 1, 2- or 3-year holding periods), we calculate how many of the initially selected funds remain a top fund based on the same performance measure. We also evaluate if the selected funds remain a top fund based on all of the other performance measures. Table 2 illustrates results for Alpha (Higher), Excess Return (Higher) and Return (Higher) as the objective/evaluation criteria (where the results of all other categories and performance measures are available upon request).
Final percentages for the objective/evaluation are based on the averages. Note, over the evaluation period (from 2000-01-31 to 2022-12-31) if the fund had a track record lower than the historical evaluation period, the measure was evaluated from its inception.
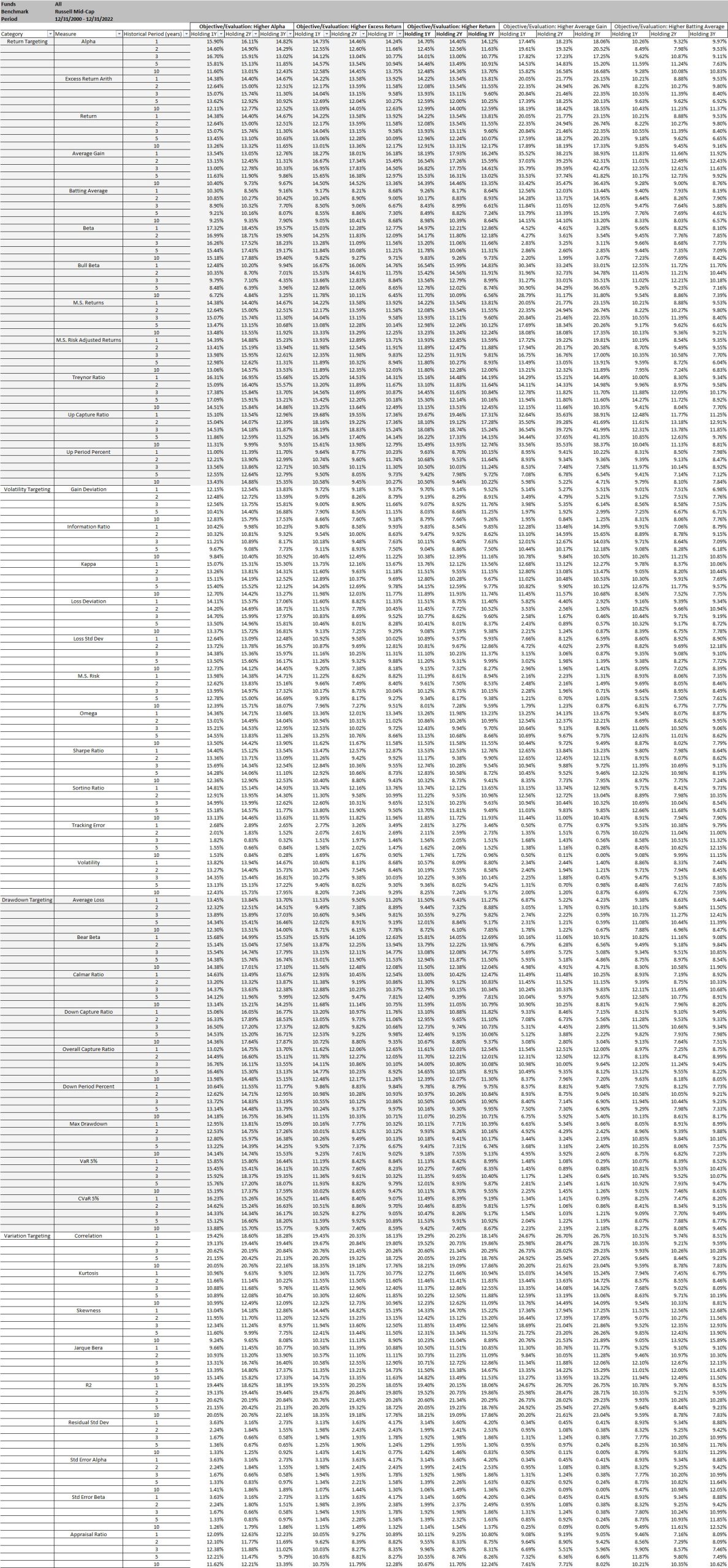
Figures 9 – 11 give the rolling numbers for the data in Table 2.
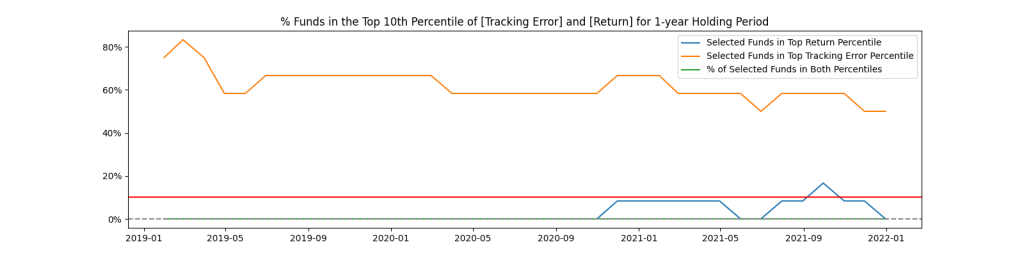
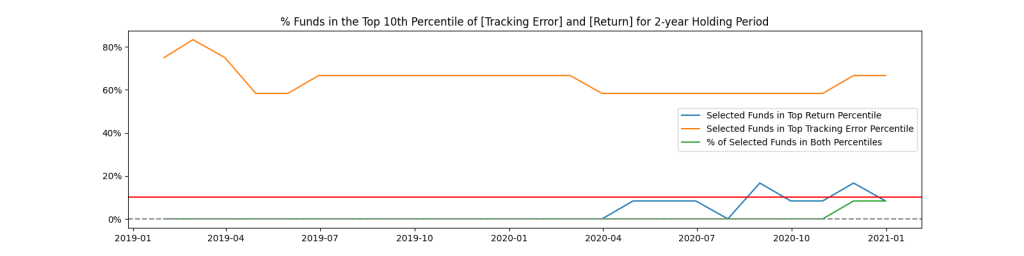
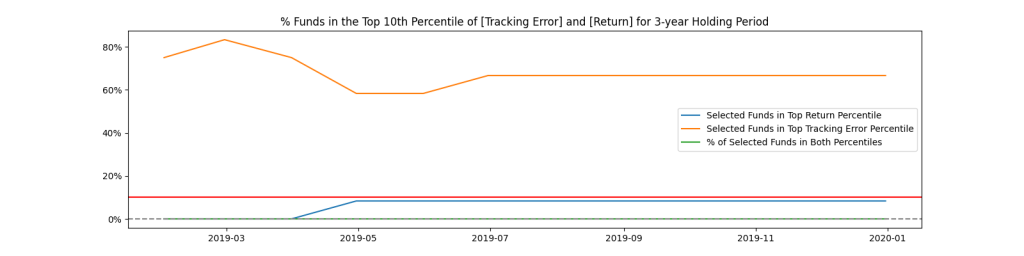
From Table 2 and Figures 9 – 11, depending on the performance measure selected there can be an up to 20% chance of being in the top decile (under certain selection criteria, evaluation criteria and investment period). Further, it should be noted that it does not imply that it is the same funds that remain top quartile. It should be noted that using a combination of fixed performance measures and weights should generally give results within the performance measure ranges.
B. Distributional Shifts
The second approach looks at the performance difference of the funds that beat the index versus the funds that did not beat the index. Herein, if certain funds did well then did their performance measures have any predictive value or show unique markers that make them better qualified for the selection. As an example, in Figures 12 we compare the distributions of funds that generated Excess Return over the index (at the end of the investment periods) with the funds that did not beat the index. Excess Return distributions may not be a clear discerning marker.
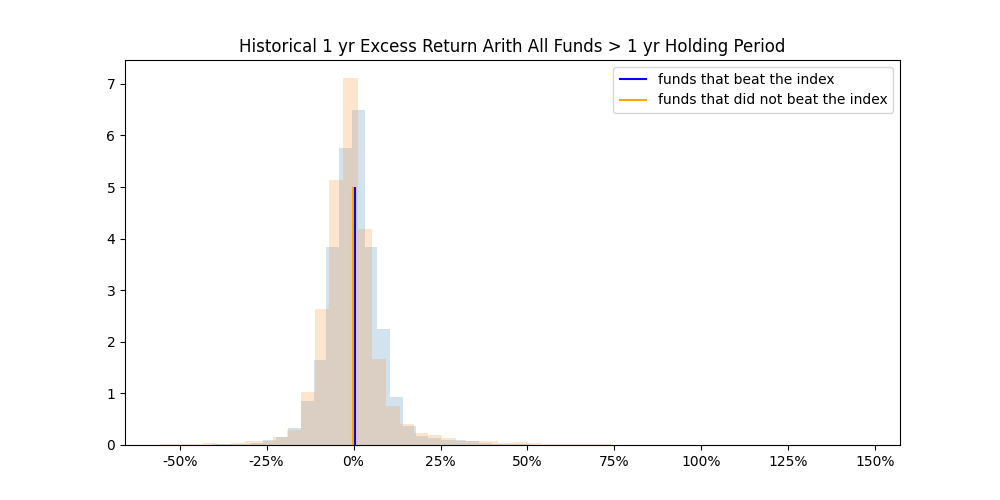
To try to isolate the discerning markers we use a distributional shift transformation to select from all the features and time periods where funds that beat and do not beat the index differ the most. Distributional shift produces a score between 0-1 of the separation between distributions. This allows different performance measures to be compared on a standardized basis. The inputs of distributional shifts are the means and standard deviations of funds that beat and did not beat the market. The larger the separation between distributions, the higher the score. As illustrated in Figure 13. some measures show greater variability in the distributions. Certain features of the analysis were omitted from the distributional shift analysis due to the effects of outliers on estimation. The inputs of distributional shift are the means and standard deviations of funds that beat and did not beat the index. Thus, any measures susceptible to outlier influence will likely bias the distributional shift results. Arithmetic Treynor ratio and geometric information ratio were omitted due to outlier influence.
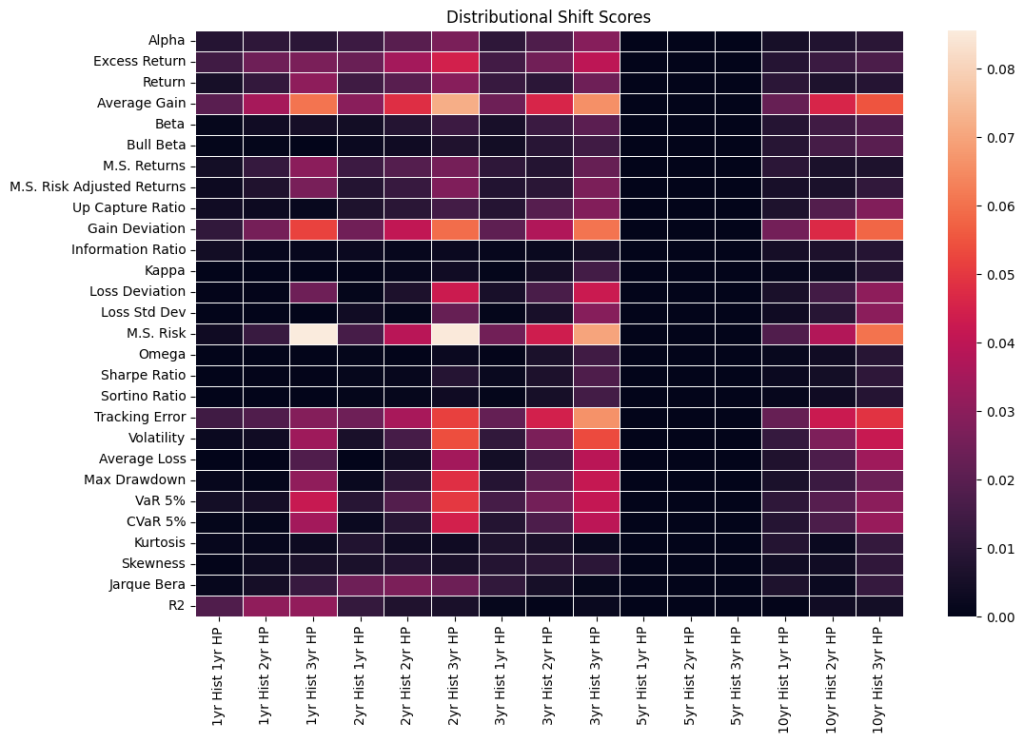
In assessing the distribution profiles of all performance measures listed in Table 1 and Figure 13 we isolate the ones that exhibit more pronounced differences (as illustrated in Figures 14 – 23).
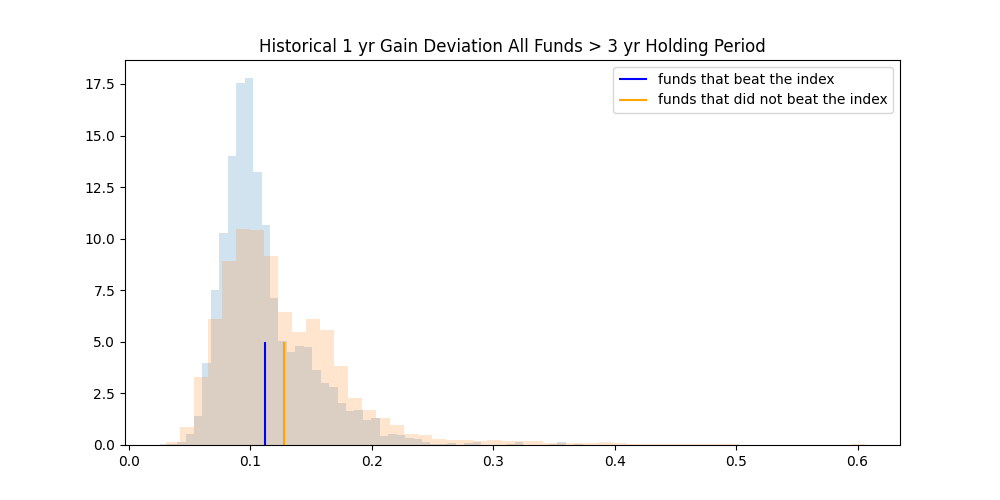
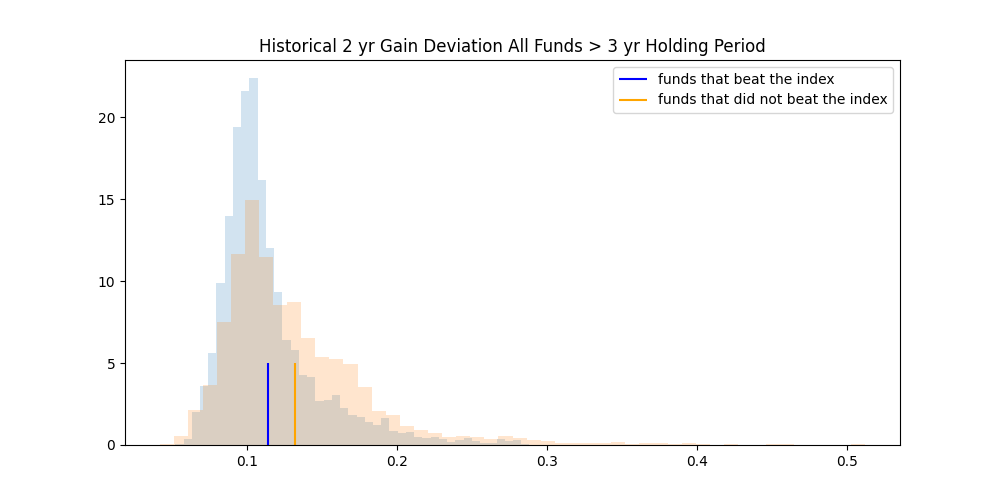
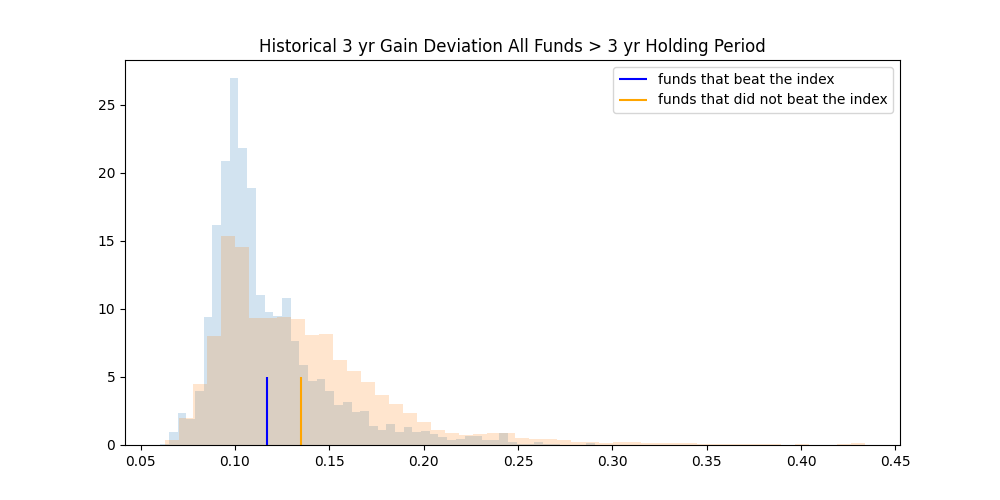
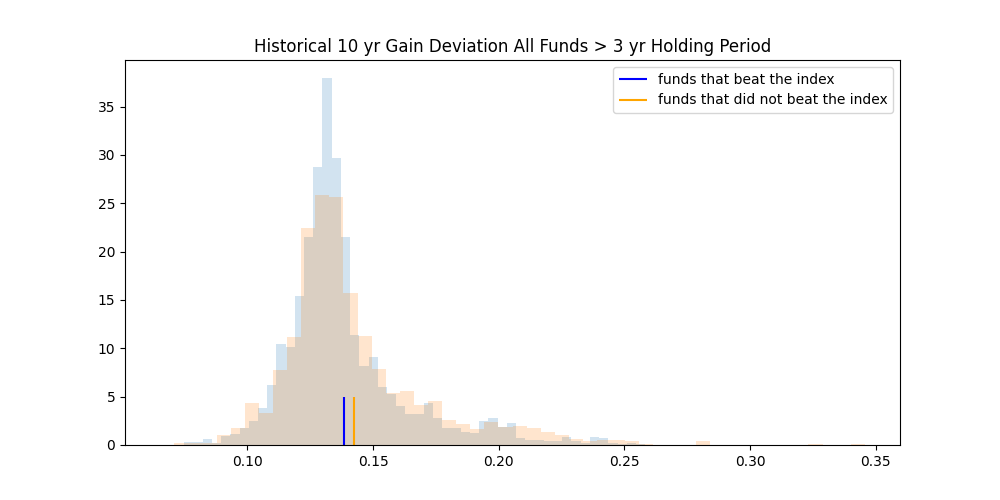
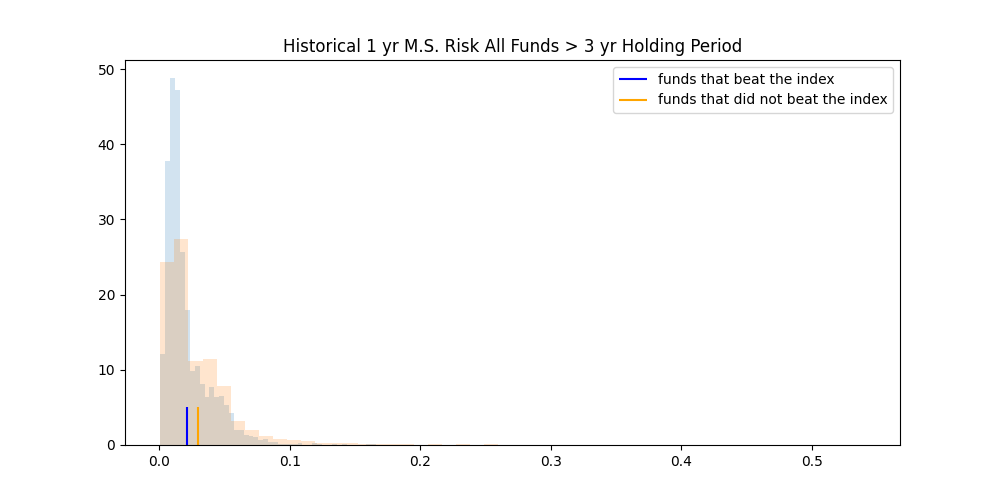
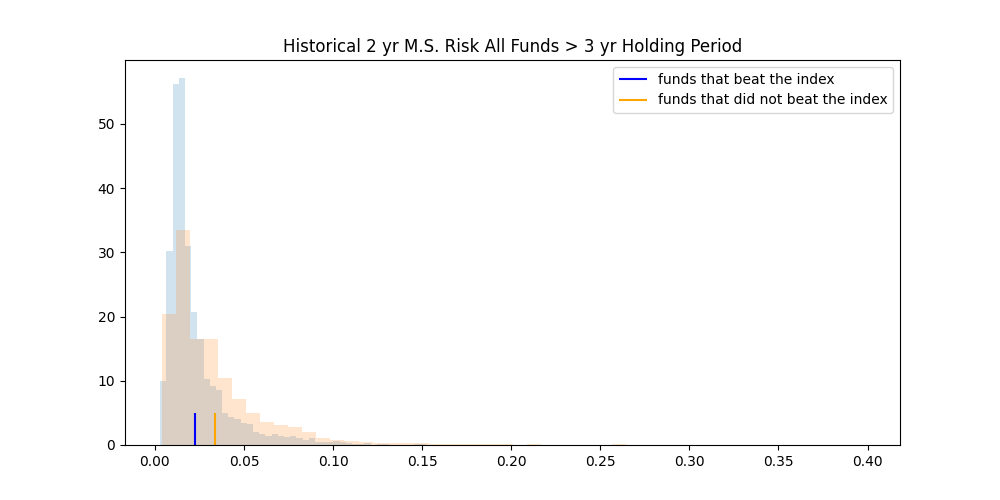
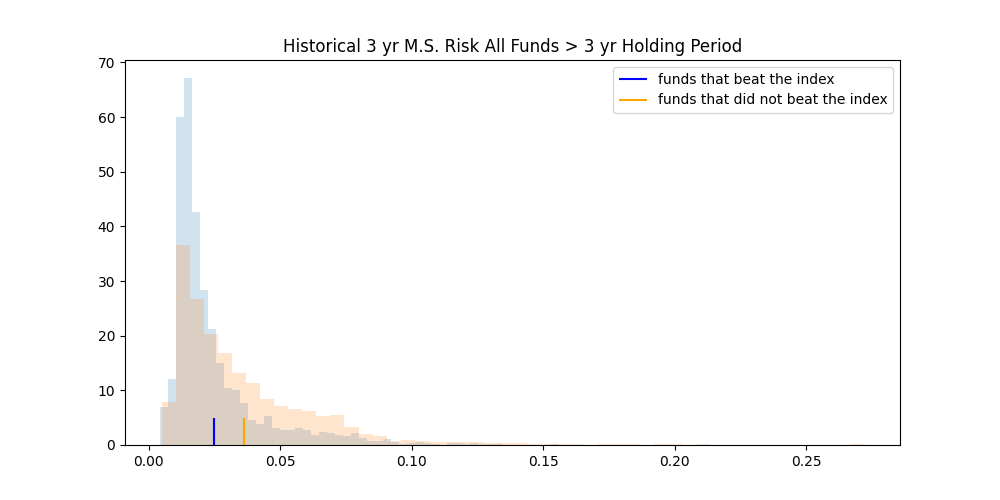
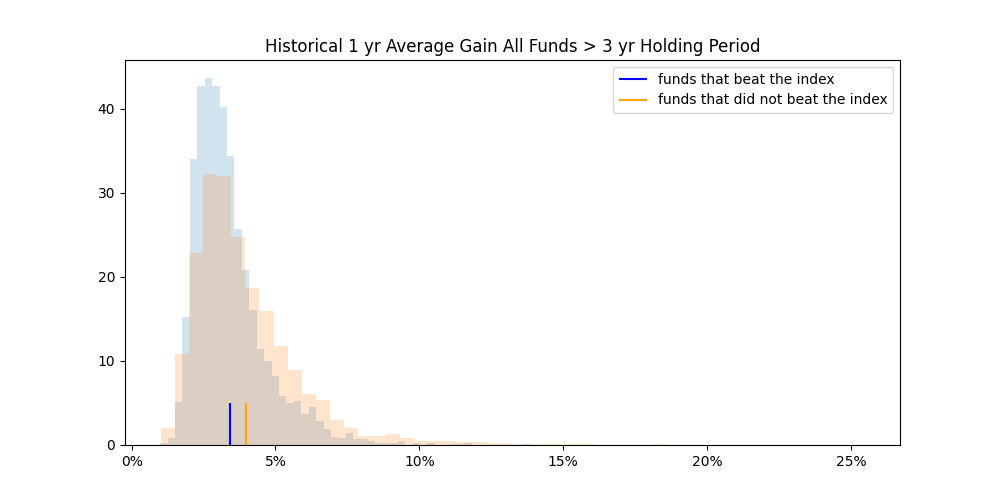
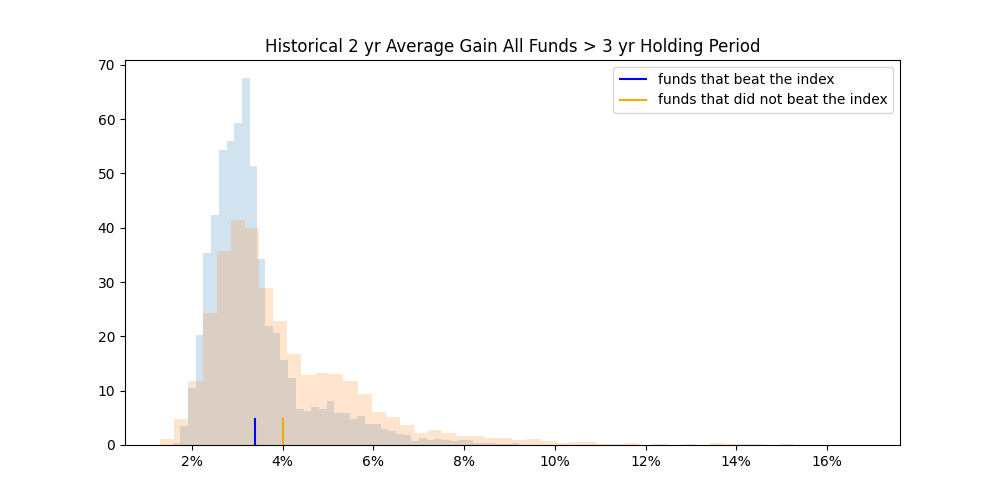
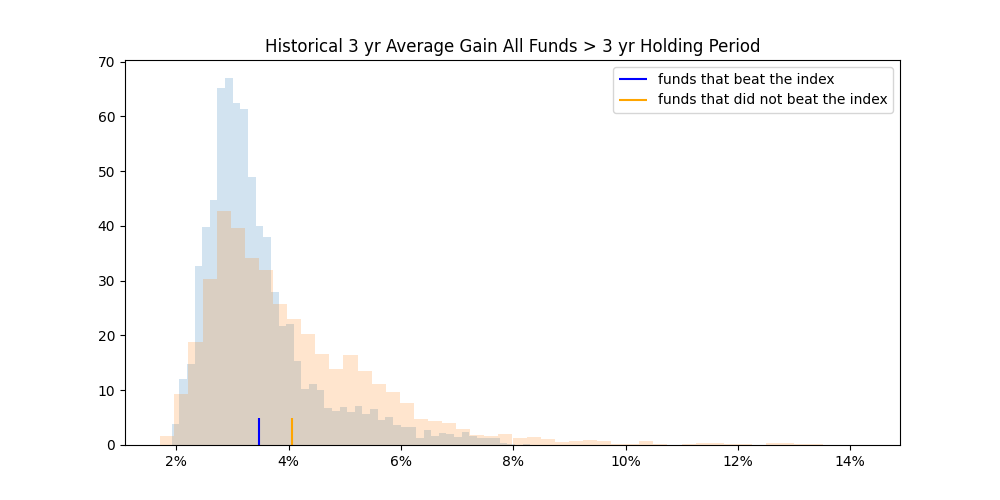
C. Regression
The third method was to run a Lasso Regression with positive coefficients and averaged over the 12/31/2000 – 12/31/2022 evaluation period. The higher the coefficient score, the more indication the individual performance measure and historical period is in determining higher fund return. As in Figures 24-26, the results of the 1, 2, and 3 year holding periods show that Average Gain, Gain Deviation, Tracking Error, and R2 performance measures score in the highest predictive power. Since the resulting coefficients are averaged over time, there could still be a high level of variability between coefficient results throughout the evaluation period.
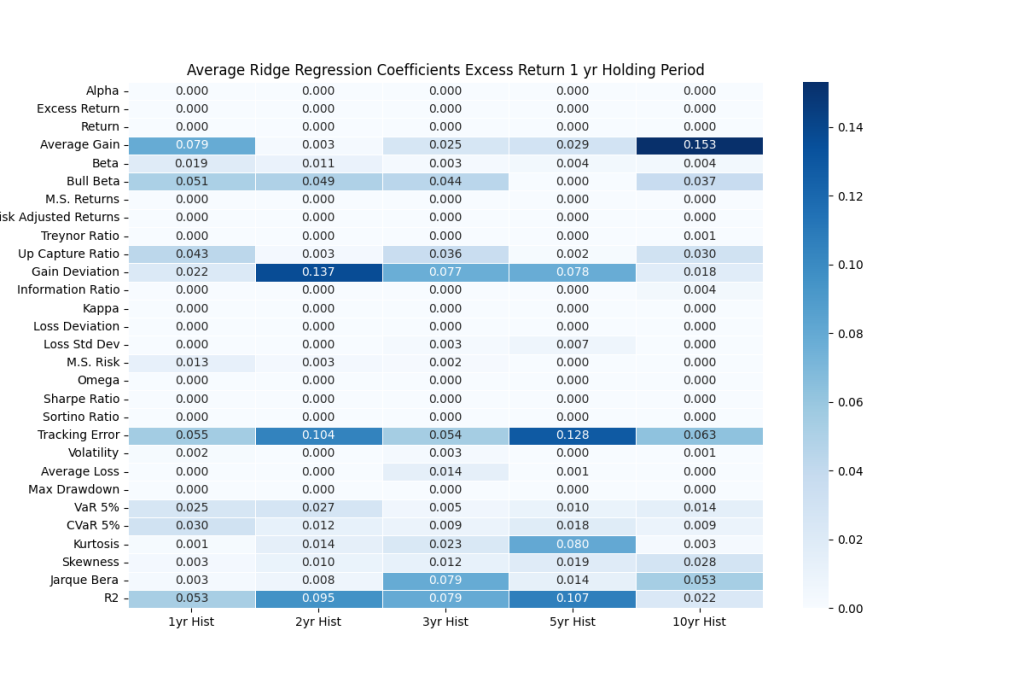
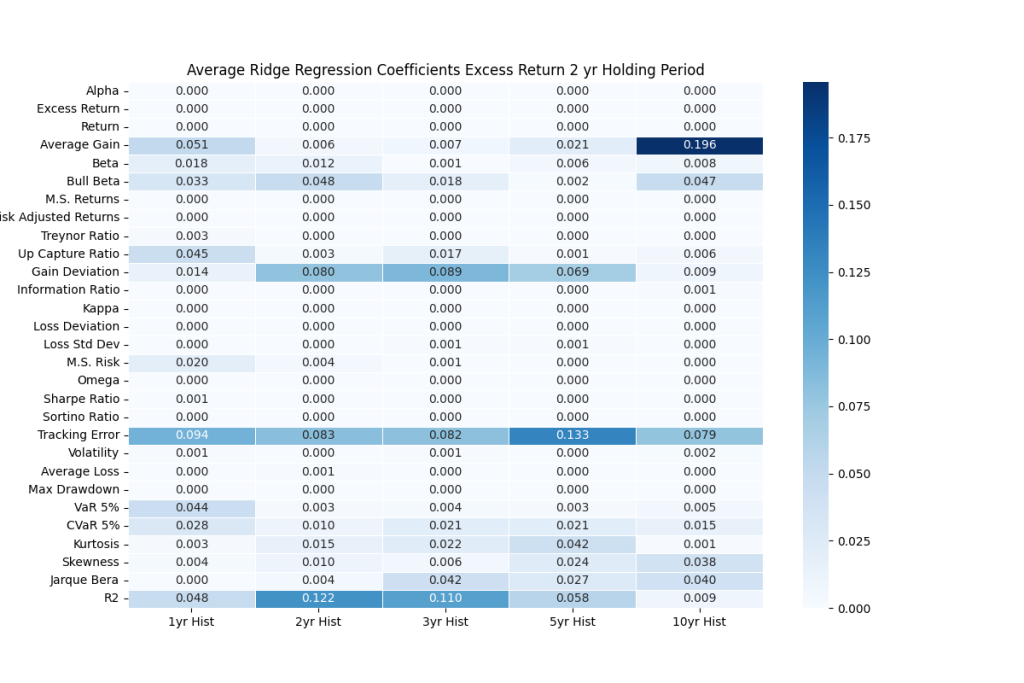
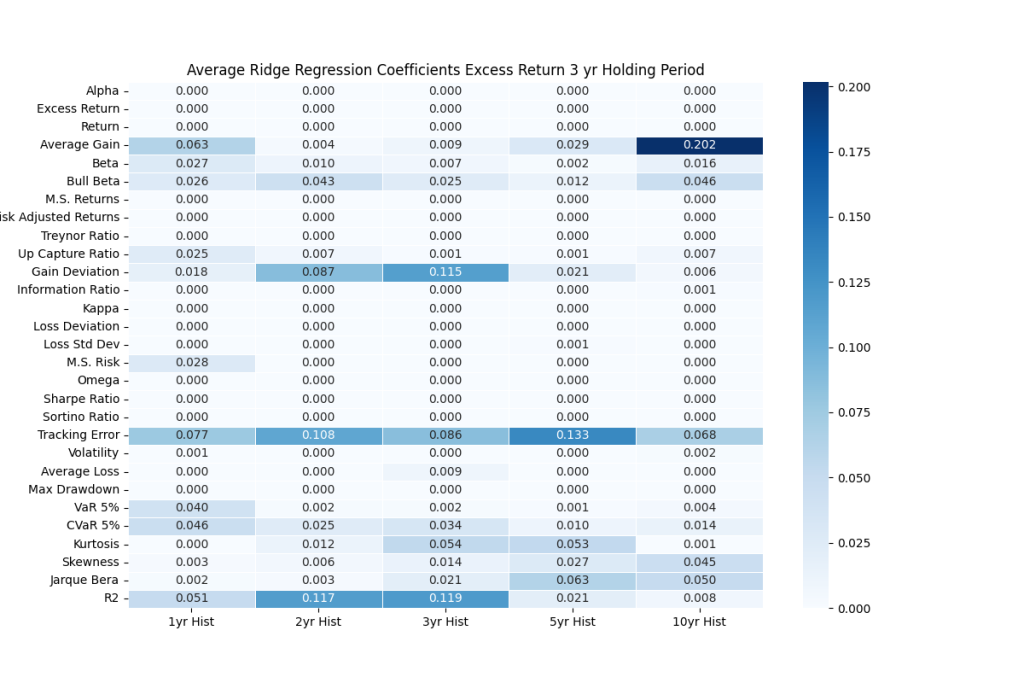
As illustrated in Figure 27, only one measure is a significant unique marker across the three assessment methods.
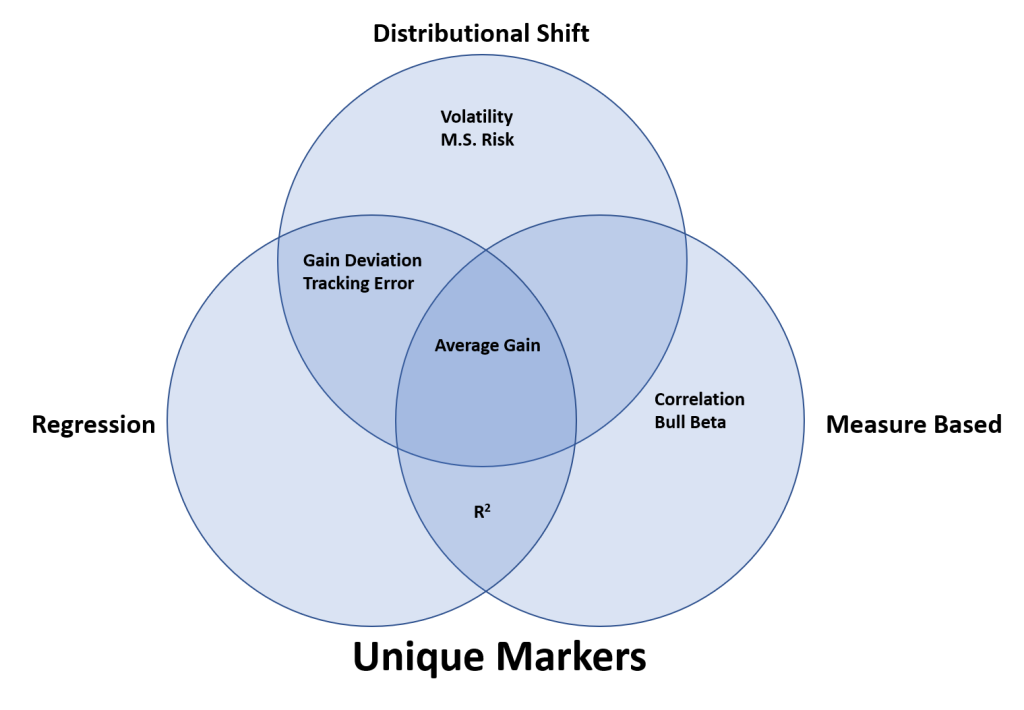
We evaluate these markers on an out of sample, rolling basis. From Table 2, assuming we would have invested in the top decile funds as classified by the historic 3-year analysis of select markers, where Figure 28, 29 and 30 give the performance of those funds after 1, 2- and 3-year holding periods using Excess Return. Note, average stands for investing equal weights in all of the identified top decile funds, worst stands for picking the worst fund in the top decile every time, and best stands for picking the best fund in the top decile every time. As a reminder, the results of all other performance measures are available upon request.
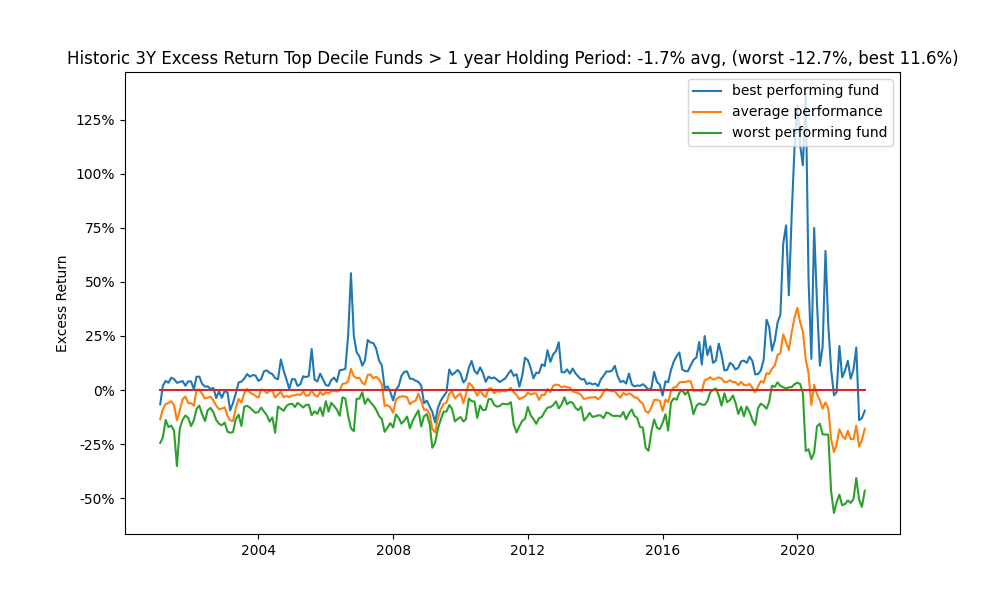
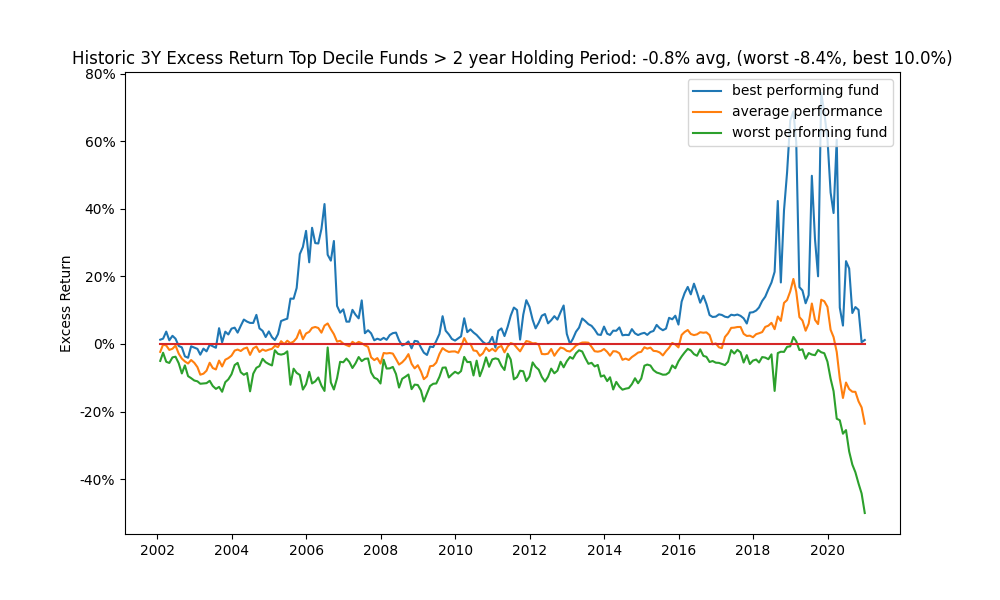
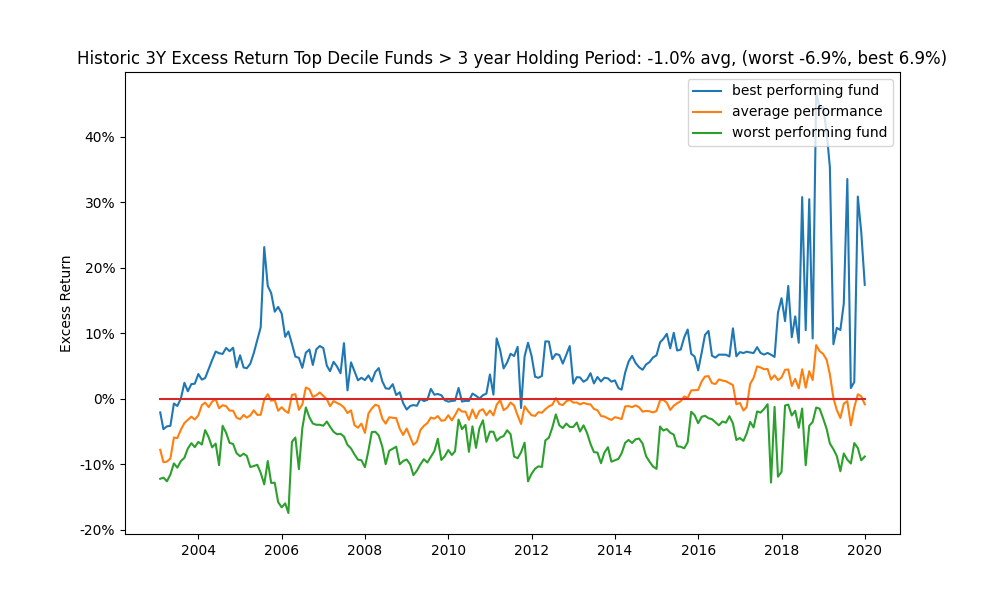
Figure 31 – 33. show the same rolling results for funds that are in the top [x]%.
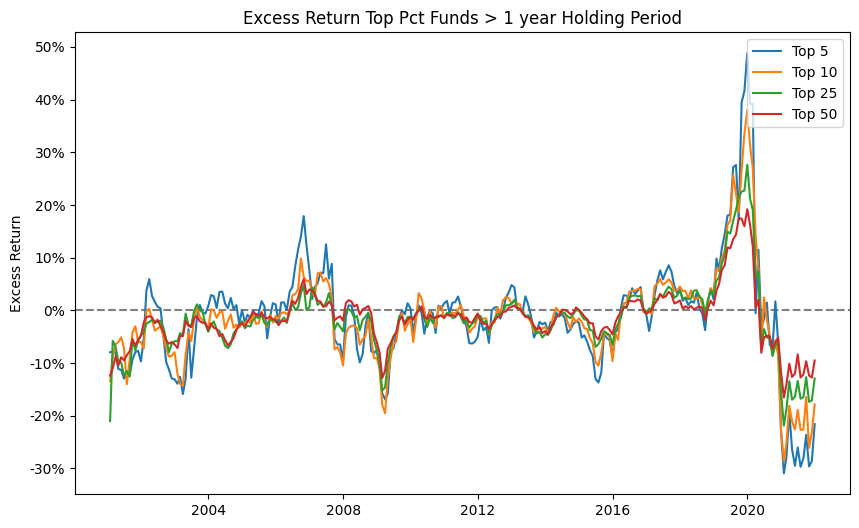
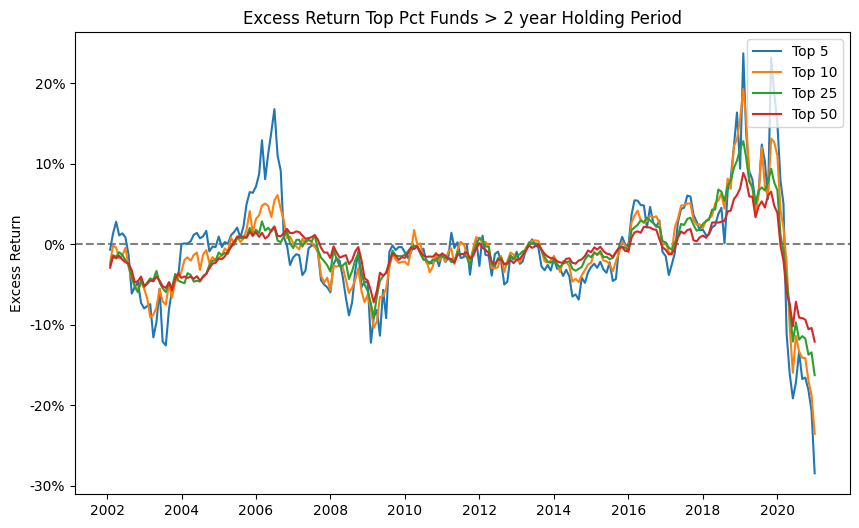
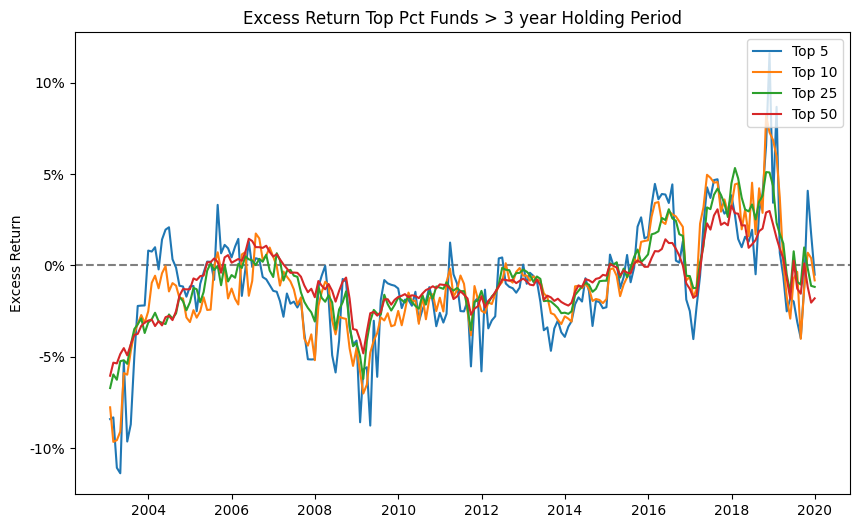
The figures above depict the Excess Return of the best, average, and worst performing funds on a rolling window basis (where historic Excess Return is the selection criteria). On average, we find that using just historic (3-year) Excess Return as a selection and evaluation criterion decreases the selection Excess Return value from the 0.46% in Table 1 to (1.7)%-(0.8)% (with a 11.6% to a (12.7)% range) and similarly for other periods (assuming impact on other performance measures are not considered).
We next look at the measures that exhibited some unique markers across the three assessments (Measure-based, Distributional shifts and Regression) to assess if they can identify the ‘better’ performing funds in the future? Since we find Average Gain to have a unique marker, we assess the Excess Returns based on using the 3-year historic performance as a selection criteria (Figures 34 – 39). On average, we find that using just historic (3-year) Average Gain as a selection and evaluation criterion decreased the selection Excess Return from the 0.46% in Table 1 to -1.2% to -1.8% (with nearly a 30% spread between the best and worst performers). Assuming impact on other performance measures are not considered. Figures 35, 37 and 39 show the results for selecting funds based on a combination of top decide funds for Average Gain and Excess Return. Here, the selection does better than randomly selecting funds, but the better performers reside within certain periods.
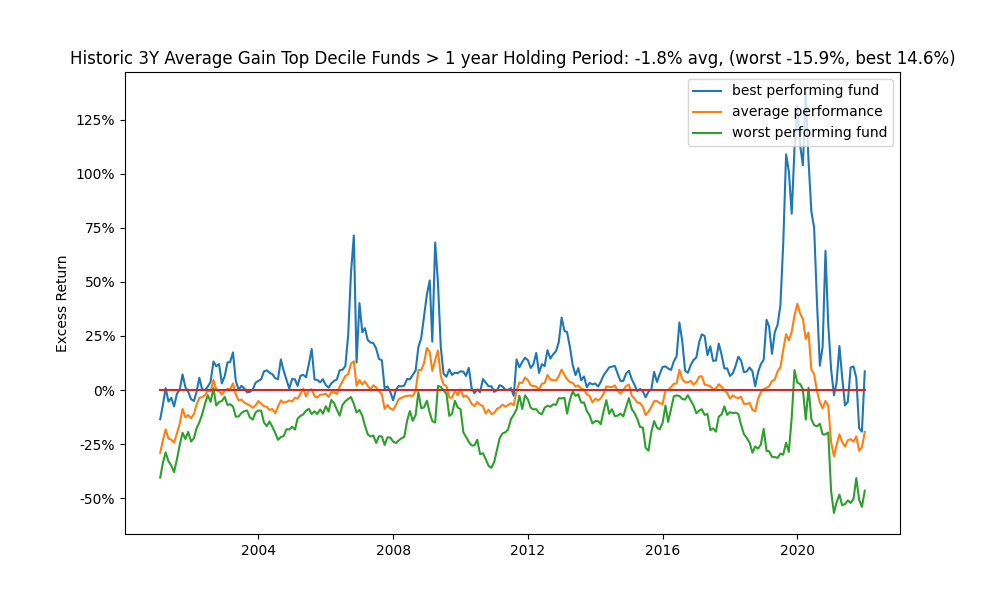
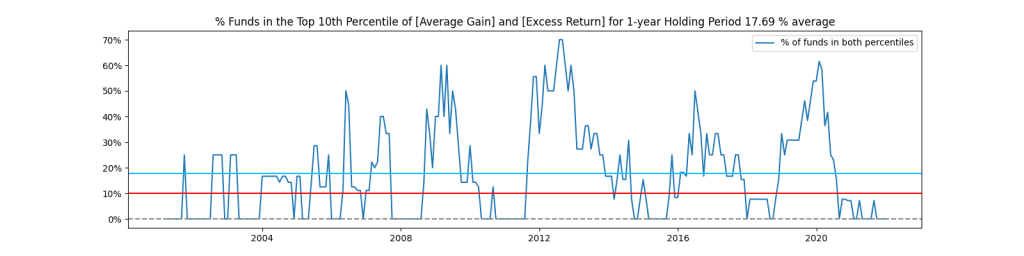
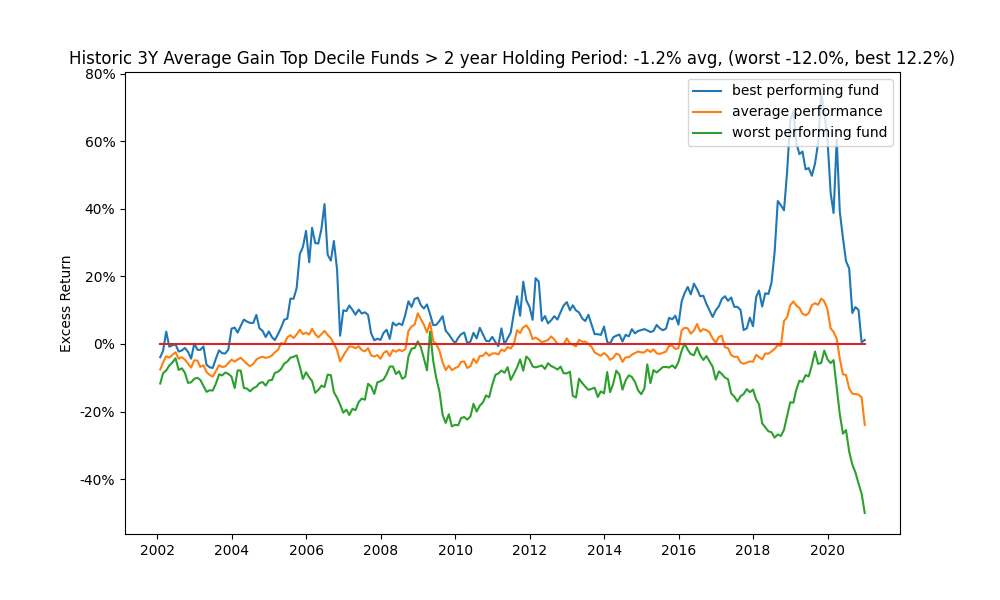
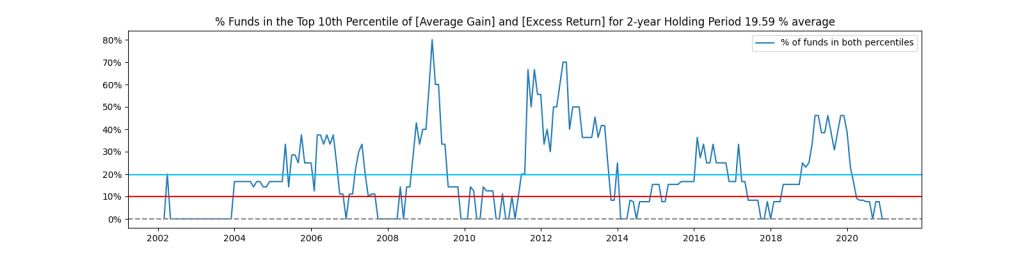
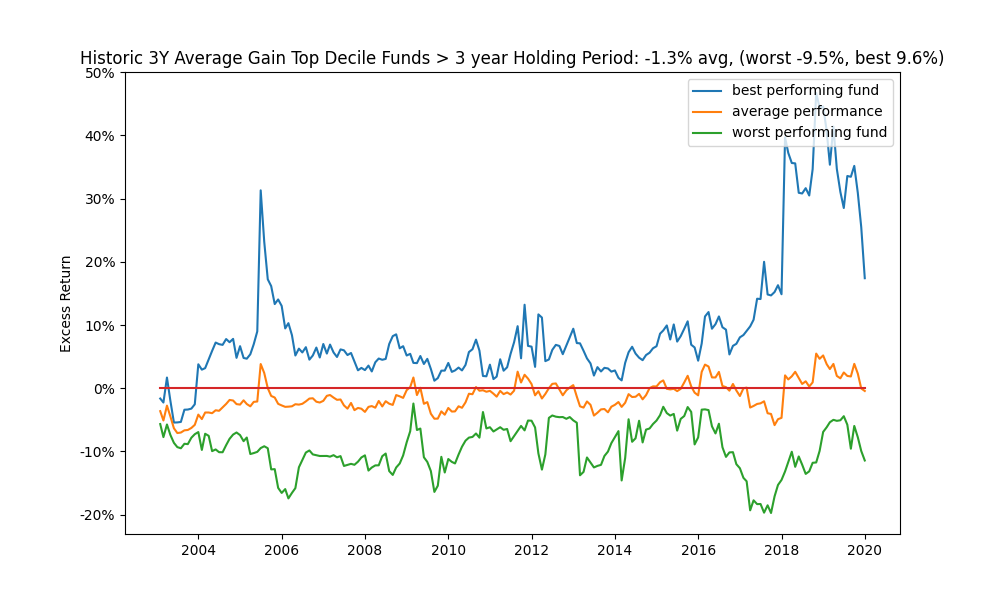
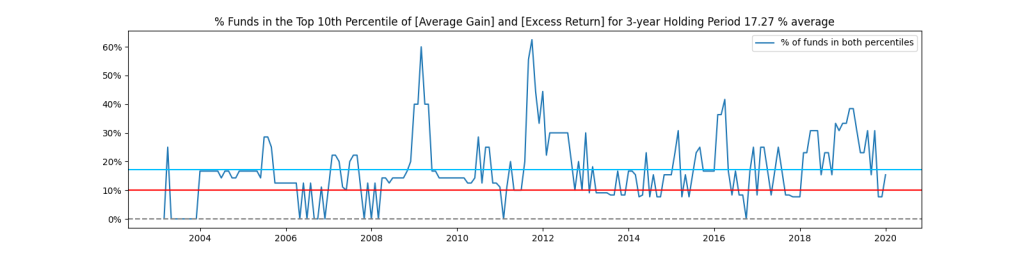
Looking at another measure Gain Deviation as a selection criteria (Figures 40 – 45), on average, the selection Excess Return value drops from -1.7% to -2.2% (again with a double digit best to worst performers spread for the presumed top tier funds). Assuming impact on other performance measures are not considered. Figures 41, 43 and 45 show the results for selecting funds based on a combination of top decide funds for Gain Deviation and Excess Return. Here, the selection does marginally better than randomly selecting funds, but again the better performers reside within certain periods.
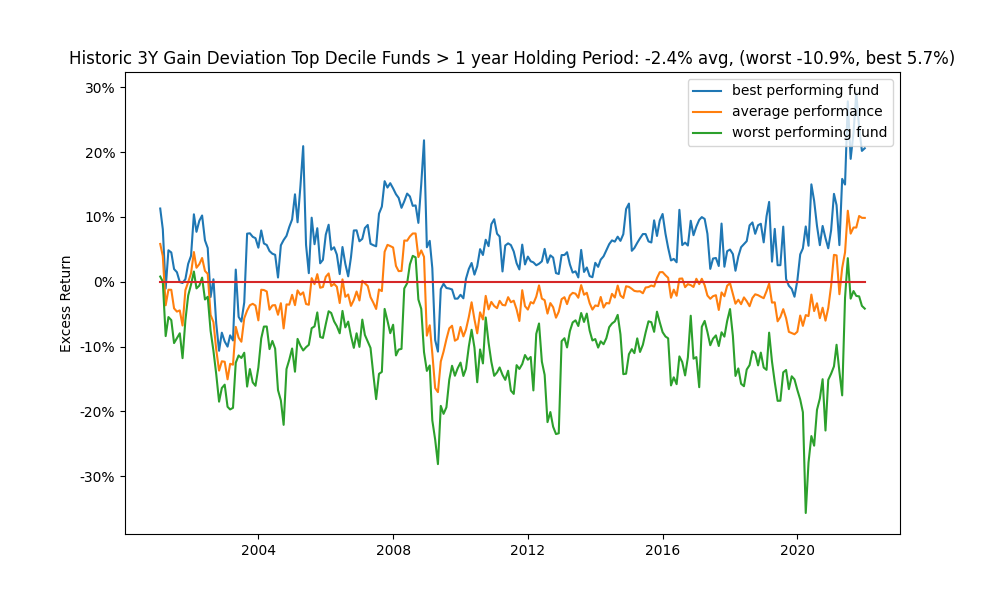
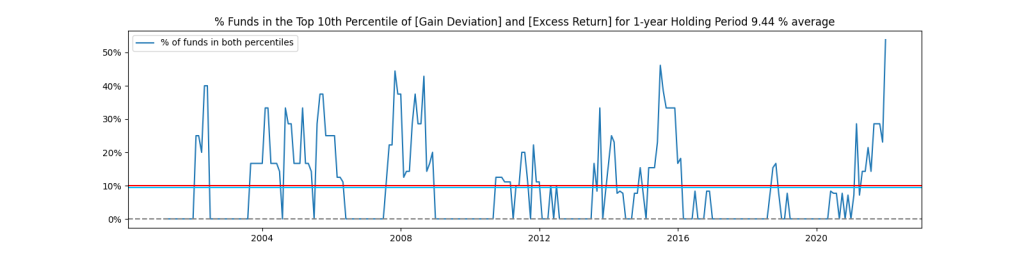
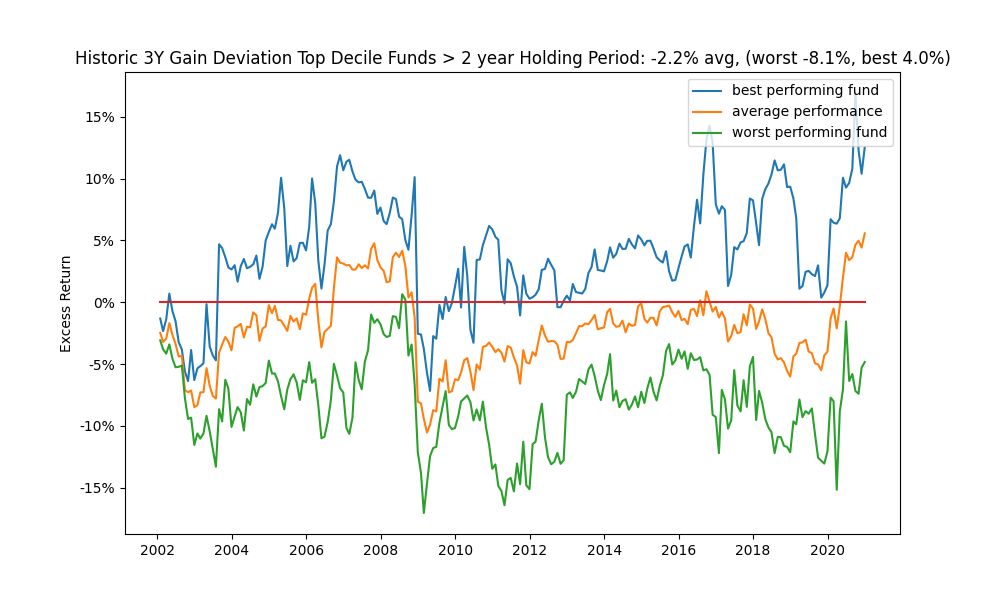
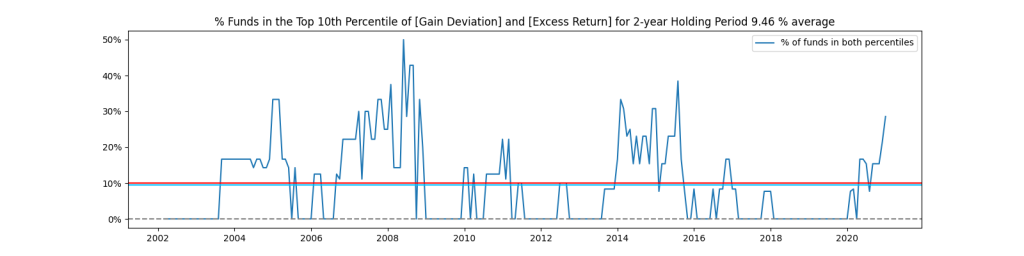
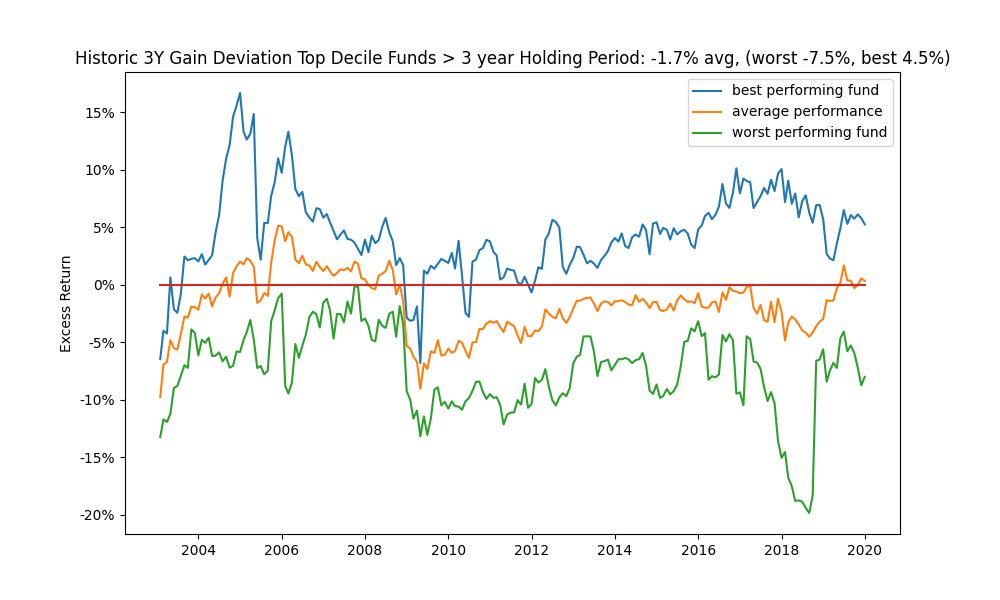
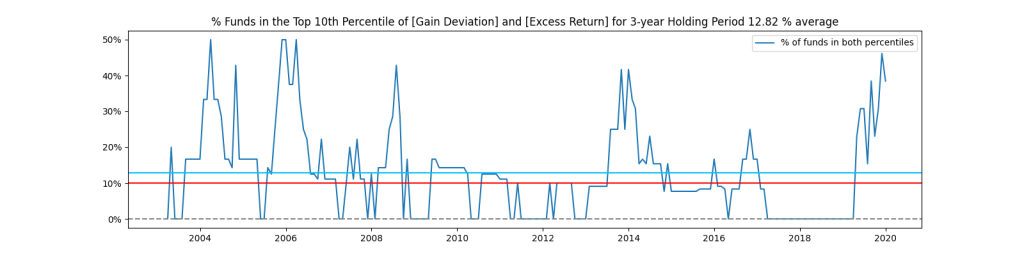
Overall, we find that none of the approaches or historic performance measures give a consistent or dependable way for isolating top performing funds over periods of time.
Fund Level. Finally we look at how the individual funds did over their 20 year history. Figure 46 column 1 shows the percentage of the times the fund was categorized as a Mid Cap fund (so consistency), column 2-4 show the percentage of times it simply did better than the index and the subsequent columns show the percentage of times it was a top tier for that performance measure.
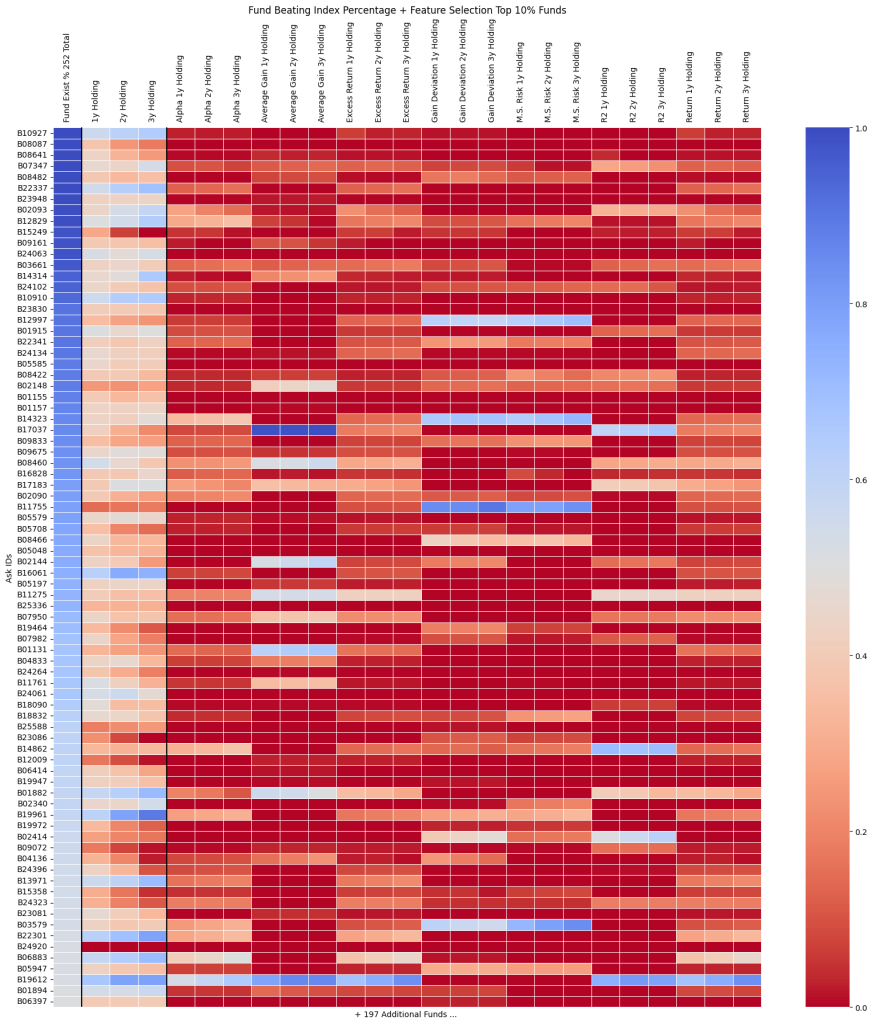
It seems there are very few pure play funds that did better than the index, but again in digging into these via the measures we find that the performance is very volatile. We cut the list to make it manageable, but information for each fund is available upon request.
US MID CAP FUNDS > FUND SELECTION (POINT IN TIME)
Looking at monthly rolling performance statistics has lots of embedded nuances, statistics and in general can be overwhelming. In this section, we do a point in time analysis, where we assume that the decisions were made on 2018-12-31 to select the top decile funds. Again starting with the 3-year historical Excess Return, we assess the Total Return of the funds in 2019, 2020 and 2021 (as illustrated in Figures 47-49).
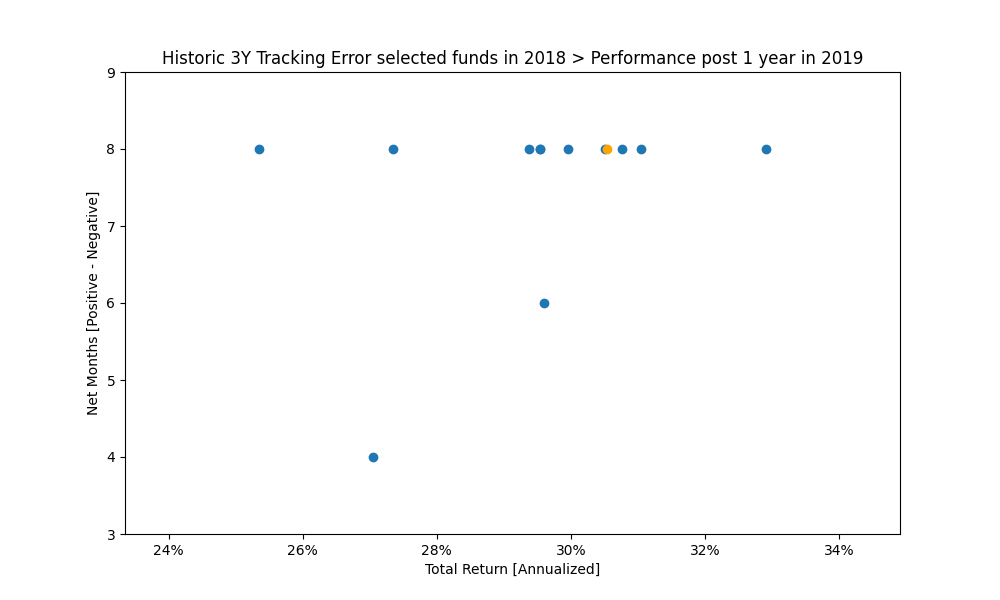
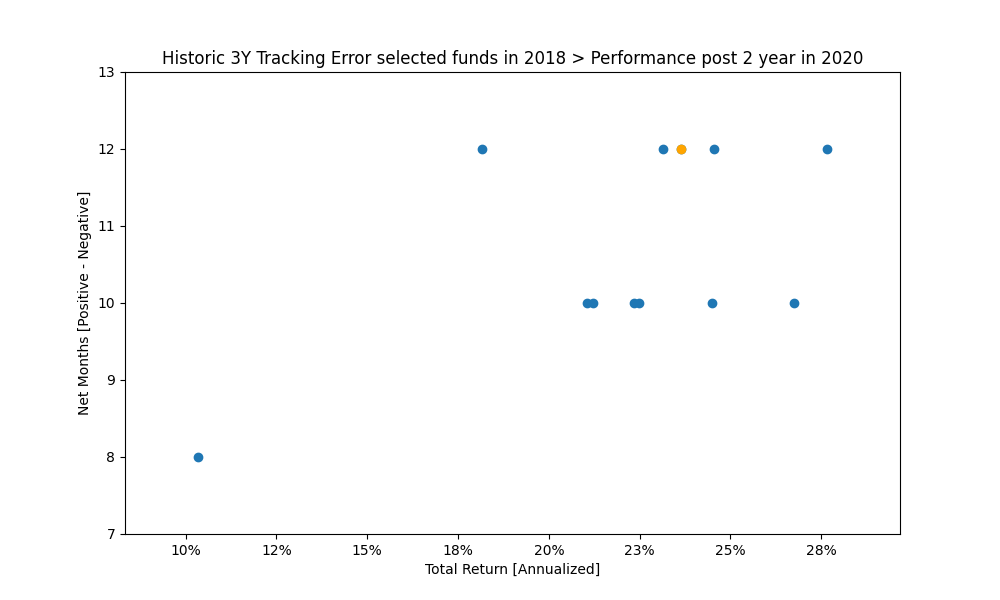
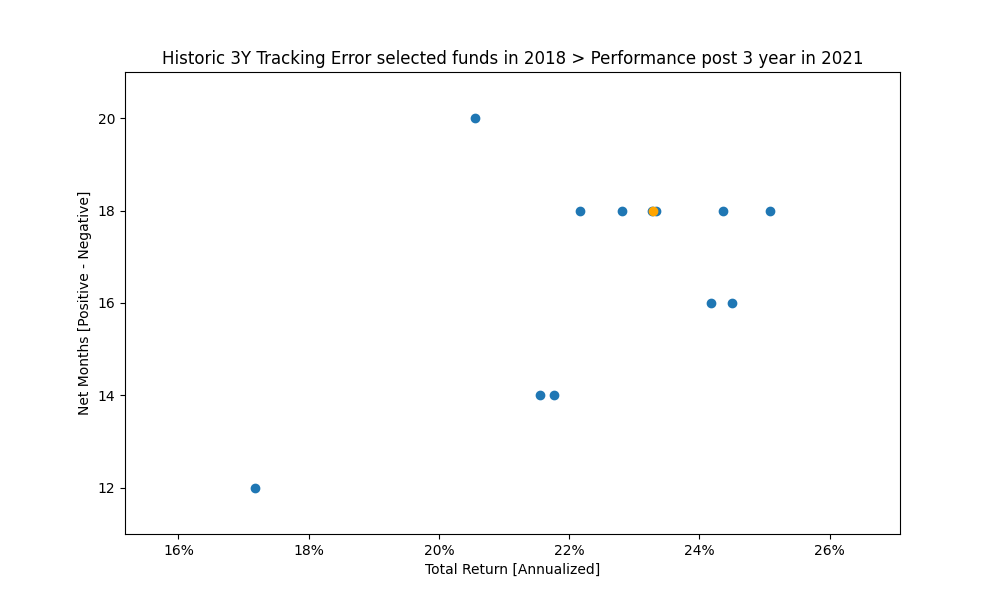
Looking at the performance of the top decile for funds selected on 2018-12-31 according to Excess Return (Figures 50 – 53) shows that as expected many of the funds did not beat the Index in the subsequent years (future 1, 2 and 3 year periods).
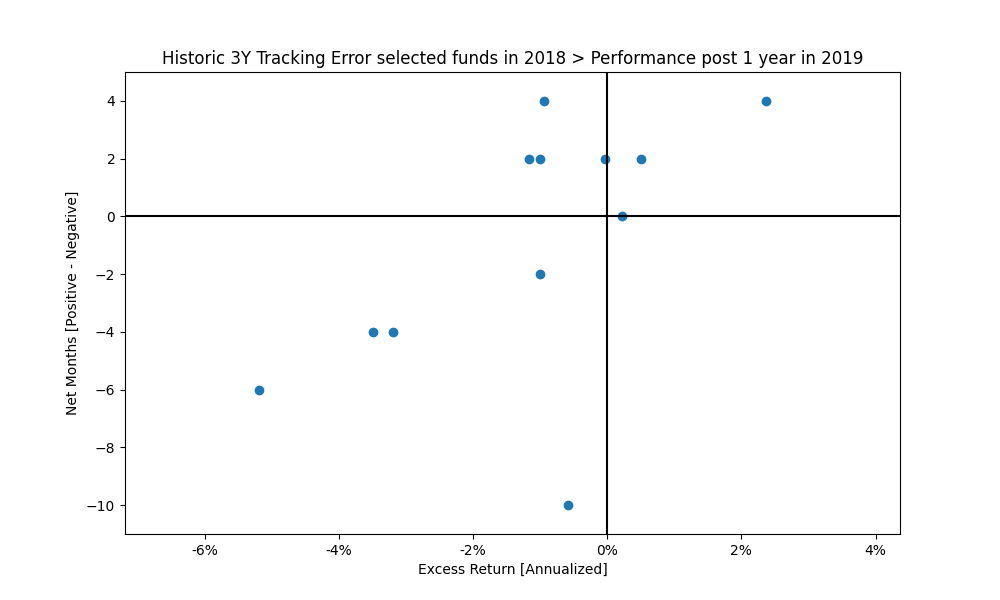
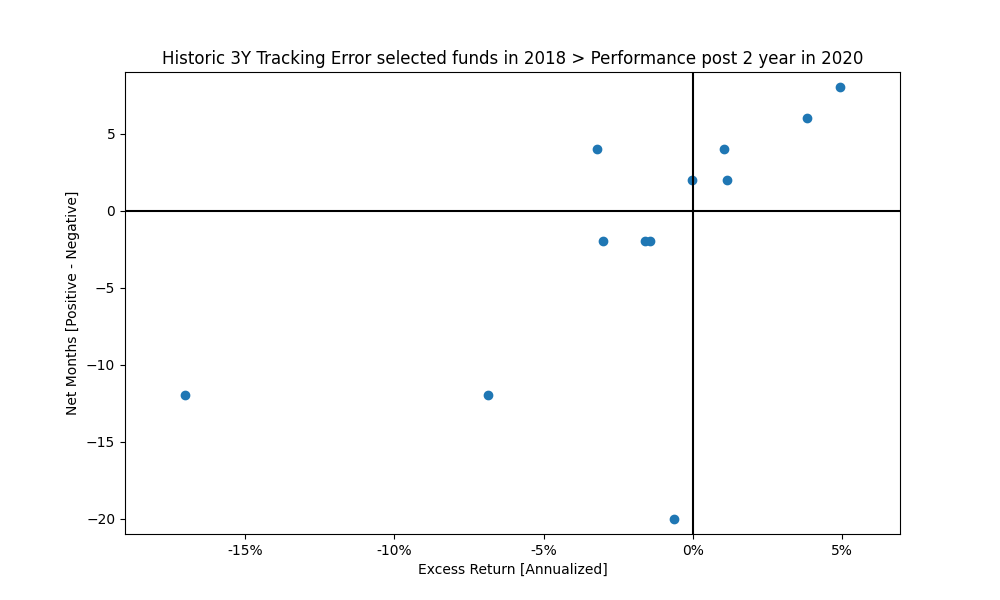
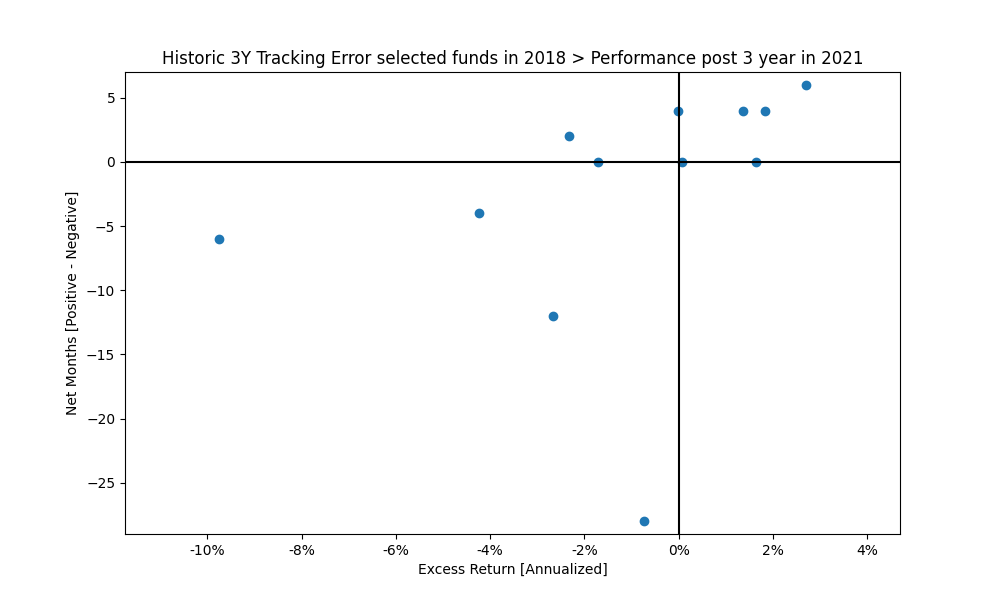
Figure 53 looks at 1 year cumulative return profile funds in the positive quartile of the funds in Figure 50. And, Figures 54 and for the funds in Figure 51. Over shorter periods the future performance expectations of even the previous top performers is extremely volatile (even on a purely Excess Return basis).
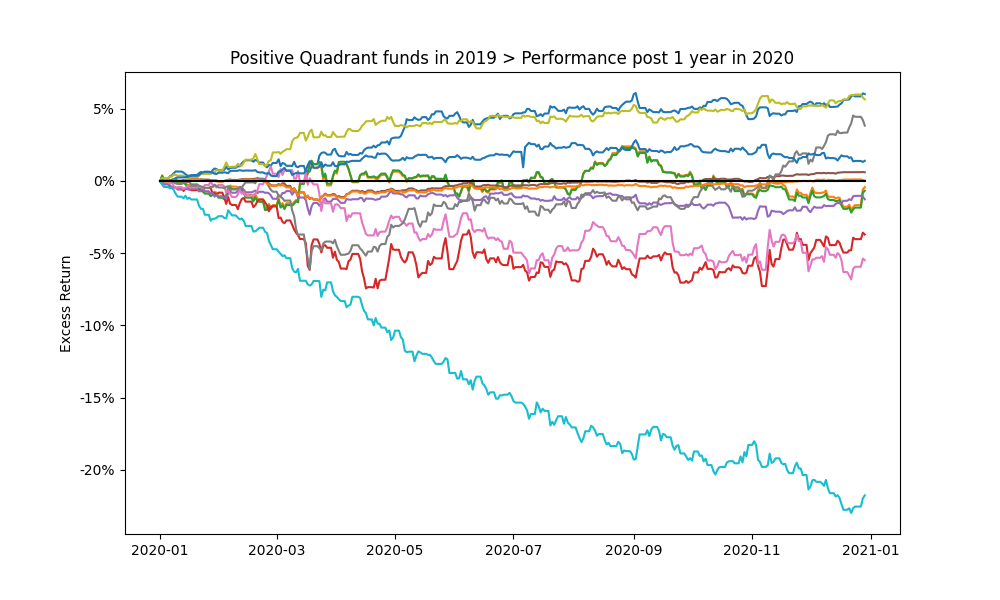
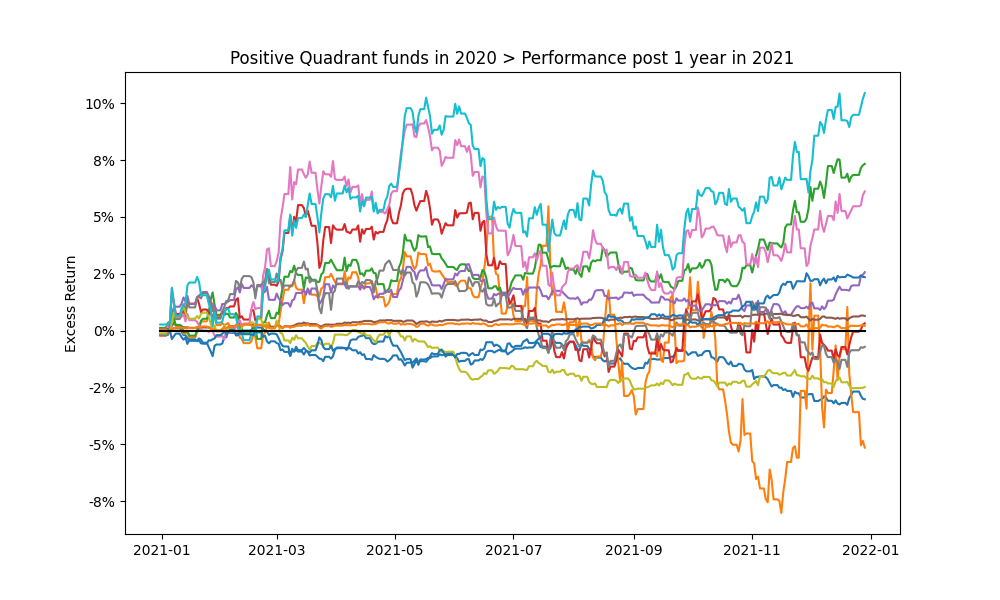
The historic baseline analysis for the US Mid Cap market indicates that investing in the Russell Mid Cap TR USD index may be a more stable bet. Deriving true value requires a lot of what ifs for isolating feature and event importance as points of entry/exit can dramatically impact the results due to the volatility shown in the analysis. The what ifs are an anecdotal, experience based or iterative process as is expected from a fundamental or historic analysis. Consequently the permutations and combinations become unmanageable really fast, which is the achilles heel of baseline historical analysis.
Contact us for information about a particular fund, performance measure, time period, etc.
Background
Insights 2.00. Mutual Fund Manager Selection – Setting up the framework
Insights 2.10. Mutual Fund Manager Selection – Basic Historic Analysis: Are you always wrong?
We begin by holistically looking at the US mutual fund manager landscape from a historical fund price perspective and assess the ability of widely used performance measures for manager selection. This is done both at the market and individual fund level. Then as simple extensions we evaluate regressions for generally fixed weighting schemes of performance measures over fixed time periods and during discrete regimes. We look at simple back testing and predefined simulations. We will give Insights for every Asset Class.
Insights 2.20. Mutual Funds – Is there value in leveraging larger datasets?
We incorporate larger volumes of macro data, market data, performance measures, holding data, alternative data, etc. We introduce forms of feature engineering to generate signals for regimes, factors, indicators and measures using both raw and reduced datasets. We also introduce synthetic data generation to supplement sparse datasets.
Insights 2.30. Mutual Funds – Machine and Deep learning edge?
We incorporate evolving market conditions, performance measures, weights, events, predictions, etc. by leveraging Machine Learning techniques for real time and simulated multivariate analysis. Then we allow the system to do feature and event engineering by assessing various Deep Learning methods.
Extensions can be drawn to other types of managers, assets and markets. Here we will stay at the framework level, but will refer to our other papers that delve into the technical nuances and discoveries. Additionally, we will share similar series of Machine and Deep Learning framework papers for other aspects of the Investment lifecycle – asset allocation, portfolio management, risk management, asset planning, product development, etc.
These are all underpinnings of our Platform, where it is built to support any/all permutation/combination of data/models/visuals.
Email: info@ask2.ai for questions.
NEXT
Insights 2.12. Mutual Fund Manager Selection – Basic Historic Analysis: Are you always wrong?
Focus: US MId Cap Mutual Funds
[1] 27,000+ if you assume all share classes. Also, not including SMAs, ETFs, etc.
[2] Hirsa, Ali and Ding, Rui and Malhotra, Satyan, Explainability Index (EI): Unifying Framework of Performance Measures and Risk of Target (RoT): Variability from Target EI (January 23, 2023). Available at SSRN: https://ssrn.com/abstract=4335455
[3] The current is based on annualized over a (x)yr period and the average is based on rolling the (x)yr window every year for 20yrs; we assess the fund/portfolio as of the last evaluation date rolled back. Analysis assumptions: Measures: Absolute. Time Variation: No. Threshold/Scale: Market Index. Categories: Yes. Weights: Equal. Type: Arithmetic. 50th percentile defined as 45-55%.